DALLE 3 Explained: Improving Image Generation with Better Captions
OpenAI’s BEST text-to-image model to date!
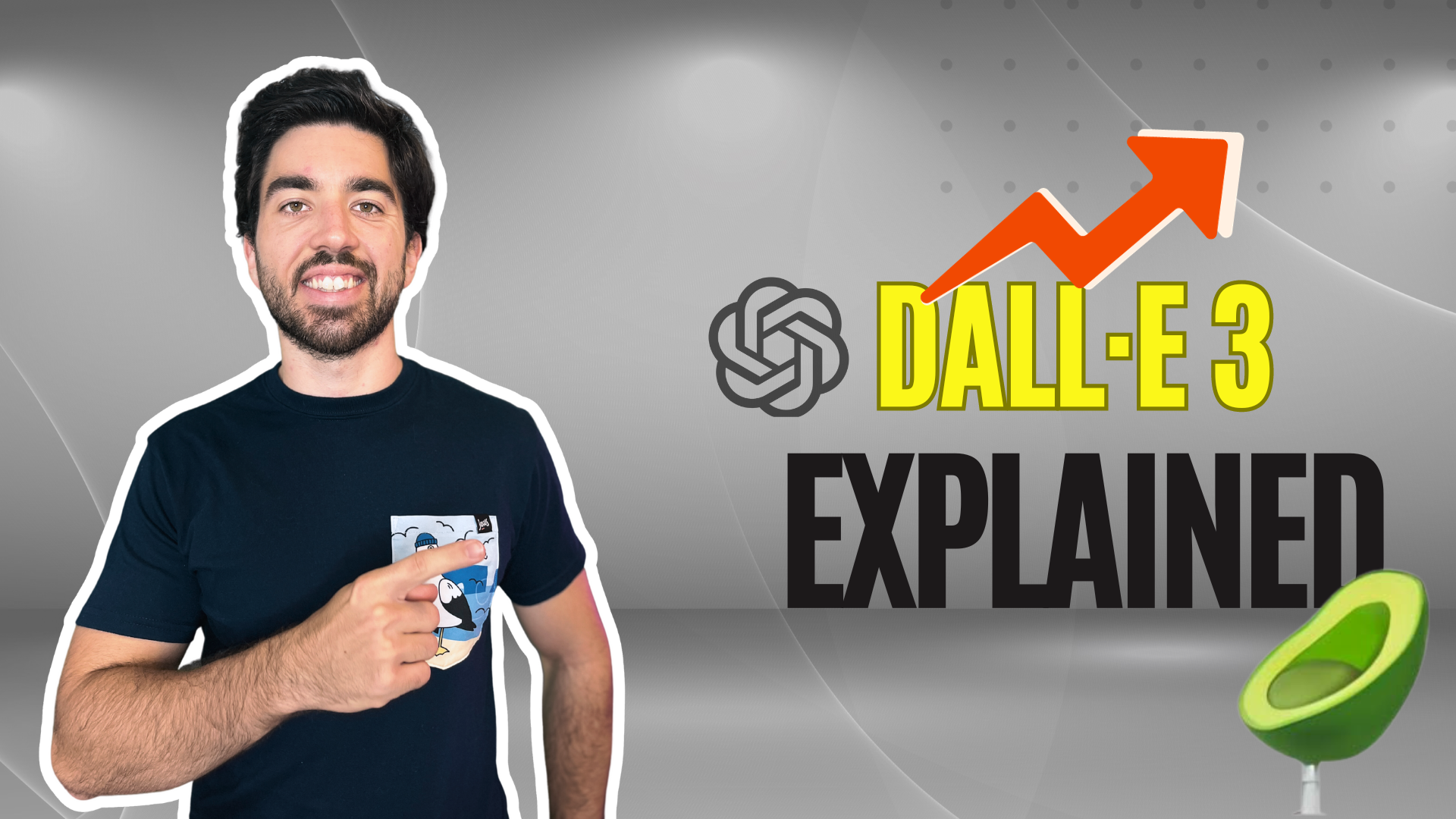
Watch the video:
Last year, we were blown away by DALL·E 2, the first super impressive text-to-image model by OpenAI. But today, prepare to step into a world where art and technology merge like never before with its third version!
Let’s dive into DALL·E 3 with a brand new paper OpenAI just released and uncover the advancements that set it leagues ahead of DALL·E 2!
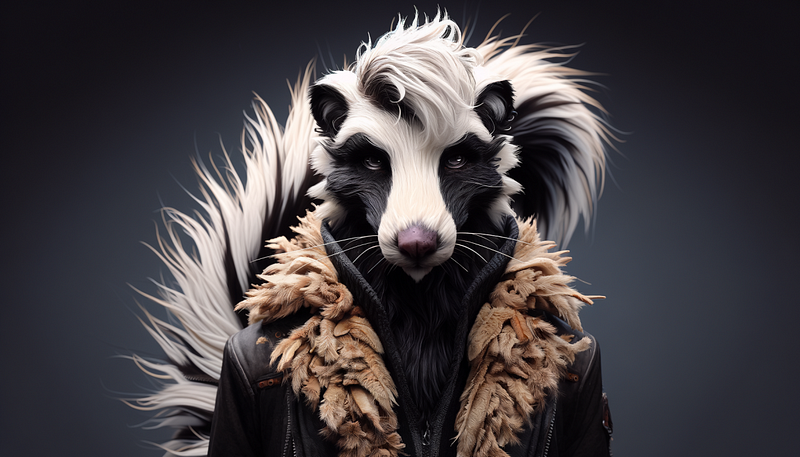
Trained on highly descriptive generated image captions, DALL·E 3 doesn’t just follow prompts — it breathes life into them. The results are incredible, and it not only understands prompts but it also understands the story behind your prompt. The progress since 2020 is just unbelievable.
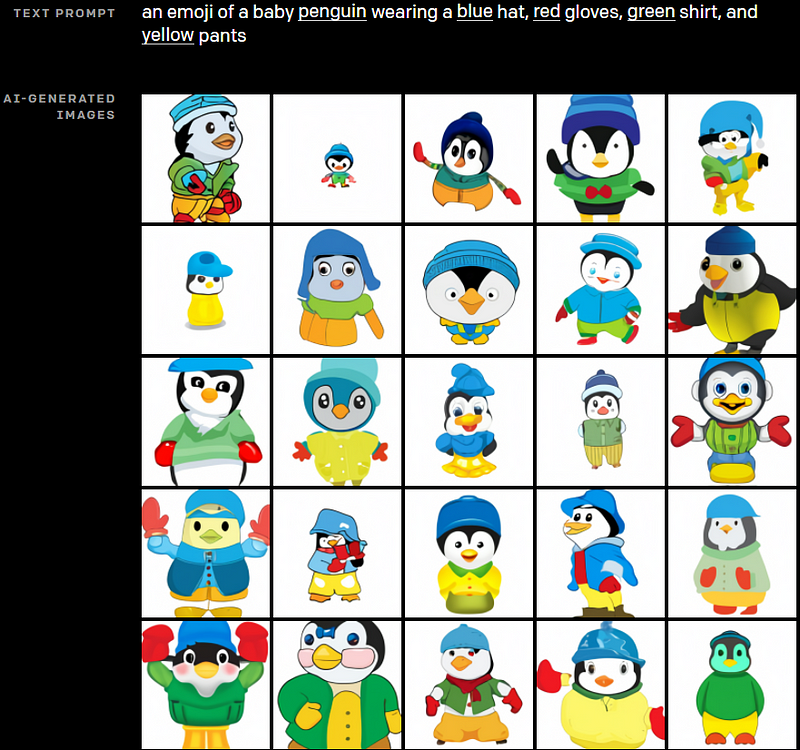
At the heart of DALL·E 3’s prowess is a robust image captioner. It’s all about the image captions, so the text fed during its training, along with the image it should be able to generate. This new image captioner is the main factor why DALLE 3 is so much better than DALLE 2. Previous models were initially trained in a self-supervised way with image-text pairs scraped from the internet. Imagine an Instagram picture and its caption or hashtags. It’s not always that informative or even linked. The authors of the post mainly describe the main subject in the picture, not the whole story behind it or the environment and text that appears in the image along with the main subject. Likewise, they don’t say where everything is placed in the image, which would be useful information to ensure the accurate recreation of a similar image. Even worse, lots of captions are just jokes or non-related thoughts or poems shared along the images. At this point, training with such data is pretty much shooting ourselves in the foot.
What if you would instead have the perfect captions? Super detailed with all the spatial information needed to re-create it? That would be perfect! But how can we have this information from millions of pictures? We could hire hundreds or thousands of humans to describe the images accurately. Or, we could use another model to understand images and generate better captions first! Well, that’s what they did. First, craft a powerful image captioner model, then use it on your current large dataset of image-caption pairs to improve them.
DALL·E 3 used 95% synthetic captions and 5% ground truth captions, a blend that elevates it to realms of creativity uncharted by its predecessors. This ratio also leads to much better results than using fewer synthetic captions. Look at those captions (image farther below)! Aren’t they better than an Instagram quick description? They are both well-written and super detailed.
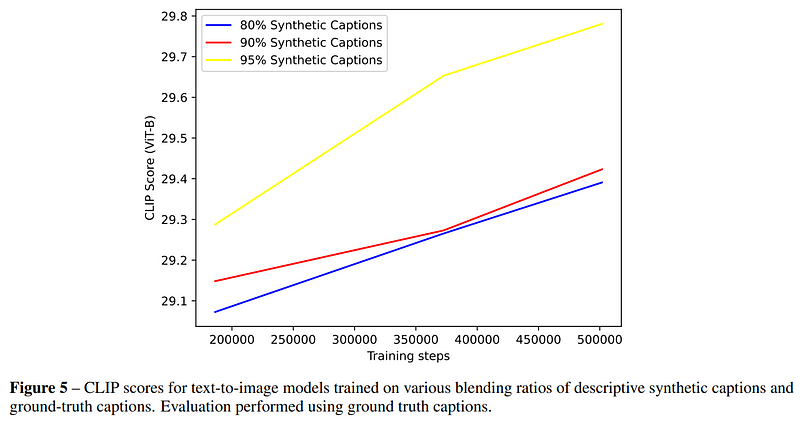
And what is this image captioner model exactly? Well, it’s pretty similar to a language model like ChatGPT. Here, instead of taking text and breaking it into tokens, we take images. Tokens are the numbers the model can understand and then process to generate sentences that would statistically make sense based on what the user asked or said. I mentioned to simply use images instead of words, but it isn’t as simple. Images are much larger than words containing thousands of pixel values. Fortunately, there are approaches like CLIP that were also trained on image-caption pairs to take images and represent them in a compressed space. It does that by converting both the image and text in a new space only it understands and ensures that both the text and image of the same pair give similar values. This means that if you then send it your image, this new representation should have a general meaning that the model can understand, just as if it would come from text. Then, to generate their synthetic data, they simply need to use this new representation to understand the images and teach to generate a good caption. This is the tricky part. Generating a good caption. Of course, they used a curated dataset of good image-caption pairs to fine-tune the model to generate better captions and not just basic ones as we had. So they still had to hire humans to build a dataset though much smaller than millions of images. They first did that with great captions about the main subject of the image to make the model understand the most important feature of the image. Then, they fine-tuned it again with not only the main subject of the image but also its surroundings, background, text found in the image, styles, coloration, etc., thus creating very descriptive captions, as you see here.
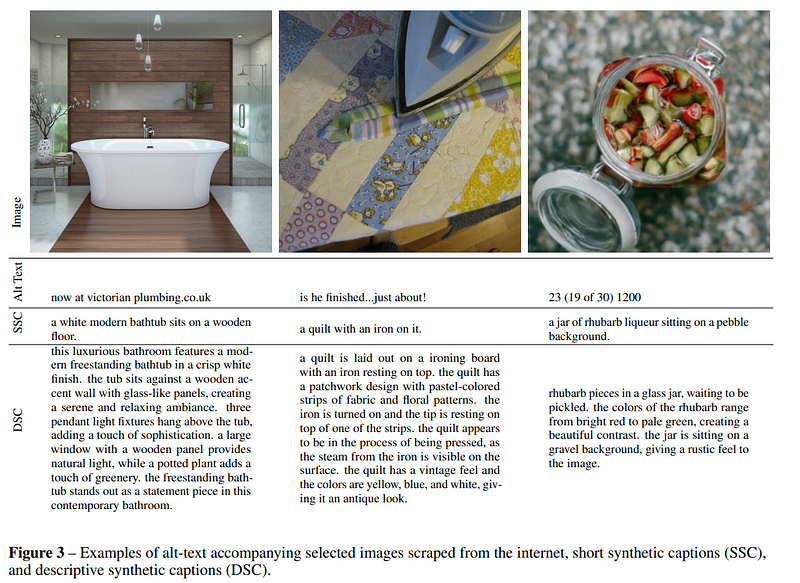
And voilà! They now have their image captioner able to take an image and generate a new descriptive caption for it! They apply it to their whole dataset and train the DALLE 3 model in the same way they did with DALLE 2, which I did a video on if you’d like more information on how it works!
By the way, if you like learning about new AI models like DALLE 3, you should subscribe to the channel since I cover most of them or even build cool products using them, which I also all share on my newsletter if you want a direct email for every new video, article, and project I share.
In evaluations, DALL·E 3 outshines DALL·E 2, with human raters consistently preferring the images generated by the newer model. It’s also much better quantitatively measured on different benchmarks like the T2I-CompBench evaluation benchmark created by Huang et al., which is a benchmark consisting of 6,000 compositional text prompts with several evaluation metrics specifically designed to evaluate compositional text-image generation models.
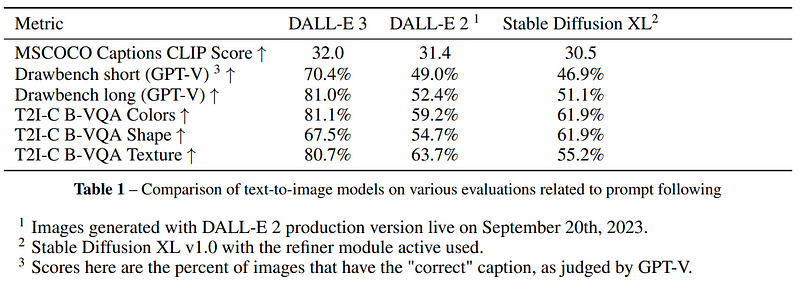
So, to recap, DALLE-3 is a huge step forward in prompt following and has amazing qualitative results, but it still has its limitations.
It struggles with image generation features like spatial awareness. It’s just really hard to have descriptive enough descriptions with location information for all objects. Also, this third version is already much better at generating text on screen, something all previous models really struggle with, but it still is quite unreliable. We will have to wait for DALLE-4 to have the proper text generated in images!
Another problem with DALLE 3 comes from the image captioner model. They have reported that the captioner is prone to hallucinating important details about an image. It often likes to give more details than less, even if it’s to create them from nothing. I guess this is just a regular behavior of LLMs, maybe because good human writers like to give details and a good story, and the model was trained on this style of writing. Anyway, there’s no complete fix on this new model hallucination problem, which is why you should always be careful when using those language models, or even image ones in this case, but if you are aware of it, you can still do wonders leveraging those models and use techniques like prompt engineering or RAG to control the model’s outputs better as I’ve shared in other videos.
I hope you’ve enjoyed this article. Again, if you’d like to dive a bit deeper into the technical details, I recommend you watch my previous videos on DALLE and DALLE-2. I’d also suggest the most intrigued of you dive into the DALLE 3 paper, where they give more insights on its creation, evaluation, and current limitations.
I’d also love to see all the creations that you can share with us on the Learn AI Together discord server; we have channels specifically for vision or random discussions. It’s been a pretty good place to get prompting advice!
Thank you for reading the whole article, and I will see you next time here or in my newsletter linked below with more amazing AI news explained!
References
- Betker et al. from OpenAI, 2023: DALL-E 3, “Improving Image Generation with Better Captions”, https://cdn.openai.com/papers/dall-e-3.pdf
- Original dalle video: https://youtu.be/DJToDLBPovg
- Dalle 2 video: https://youtu.be/rdGVbPI42sA