Impressive photo restoration by AI !
This new and completely free AI model can fix most of your old pictures in a split second!
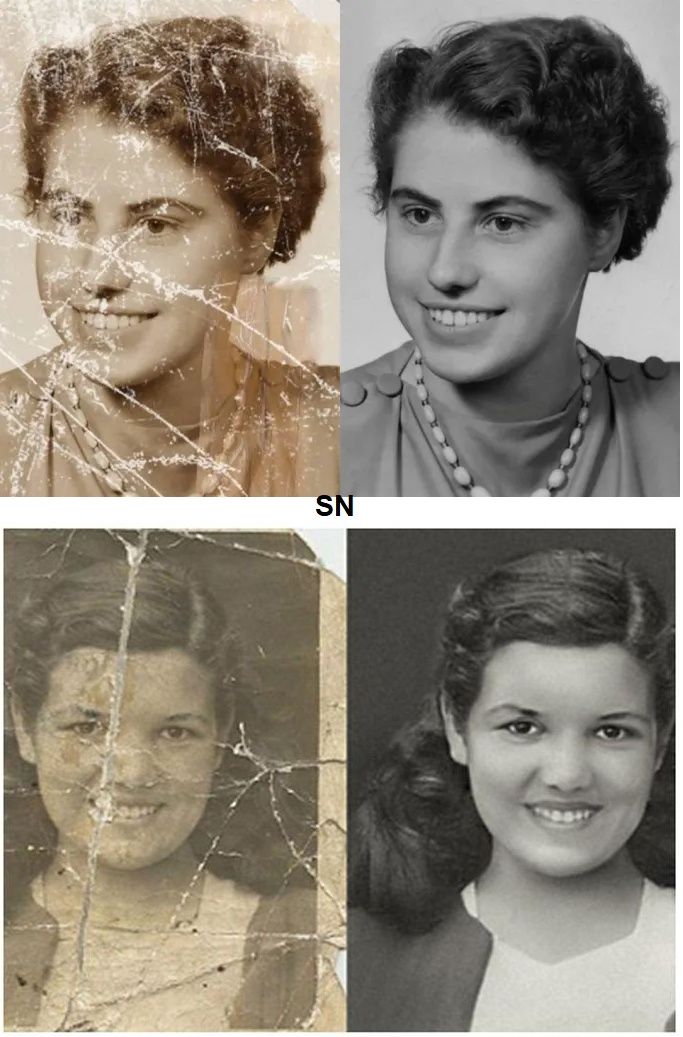
Watch the video for more results:
Do you also have old pictures of yourself or close ones that didn’t age well or that you, or your parents, took before we could produce high-quality images? I do, and I felt like those memories were damaged forever. Boy, was I wrong!
This new and completely free AI model can fix most of your old pictures in a split second. It works well even with very low or high-quality inputs, which is typically quite the challenge.
This week’s paper called Towards Real-World Blind Face Restoration with Generative Facial Prior tackles the photo restoration task with outstanding results. What’s even cooler is that you can try it yourself and in your preferred way. They have open-sourced their code, created a demo and online applications for you to try right now. If the results you’ve seen above aren’t convincing enough, just watch the video and let me know what you think in the comments, I know it will blow your mind!
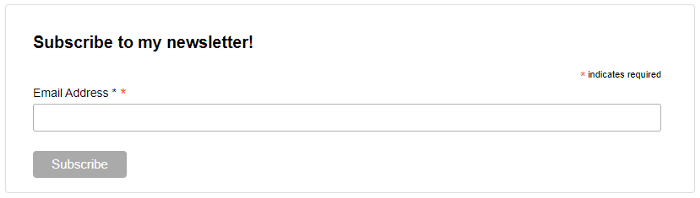
I mentioned that the model worked well on low-quality images. Just look at the results and level of detail compared to the other approaches…
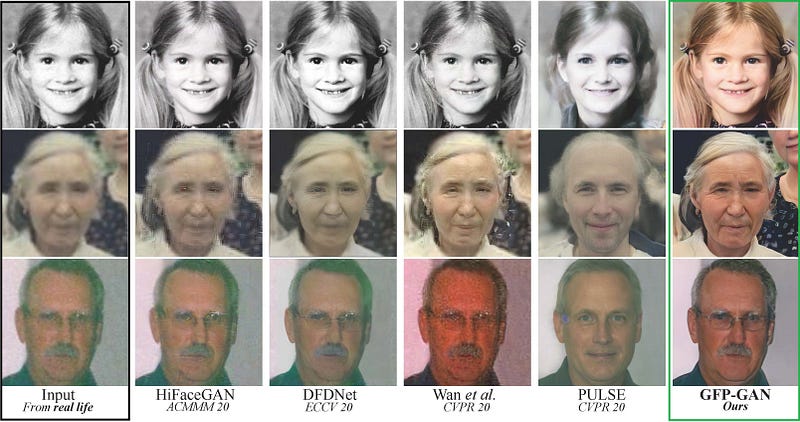
These results are just incredible. They do not represent the actual image. It’s important to understand that these results are just guesses from the model- guesses that seem pretty damn close. To human eyes, it seems like the same image representing the person. We couldn’t guess that a model created more pixels without knowing anything else about the person.
So the model tries its best to understand what’s in the picture, fill in the gaps, or add pixels if the image is of low resolution. But how does it work? How can an AI model understand what is in the picture and, more impressing, understand what isn’t in it, such as what was in the place of this scratch?
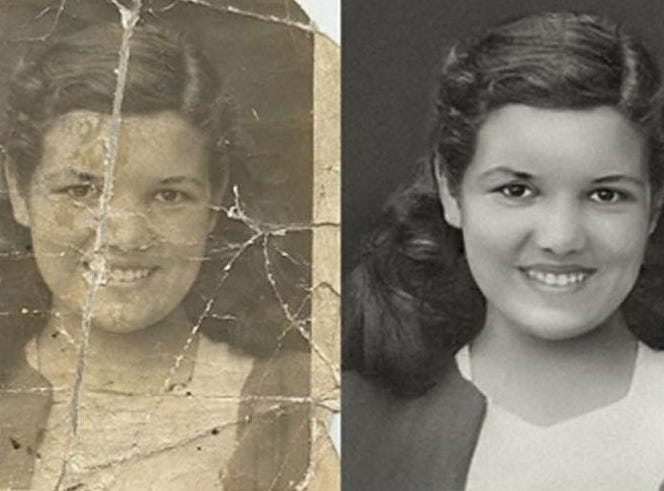
Well, as you will see, GANs aren’t dead yet! Indeed, the researchers didn’t create anything new. They *simply* maximized GANs performances by helping the network as much as possible. And what could be better to help a GAN architecture than using another GAN?
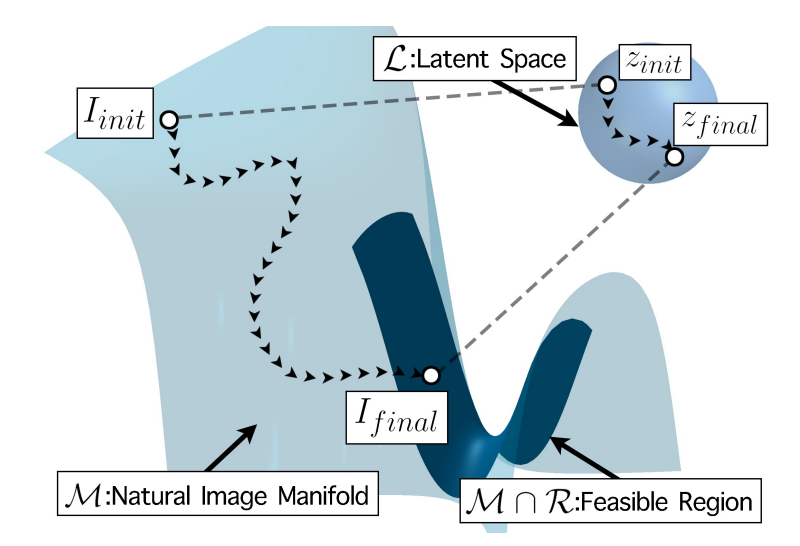
Their model is called GFP-GAN, for a reason. GFP stands for Generative Facial Prior, and I already covered what GANs are in multiple videos if it sounds like another language to you. For example, a model I covered last year for image upsampling called PULSE uses pre-trained GANs like StyleGAN-2 from NVIDIA and optimizes the encodings, called latent code, during training to improve the reconstruction quality. Again, if this doesn’t ring any bell, please take a few minutes to watch the video I made covering the PULSE model.
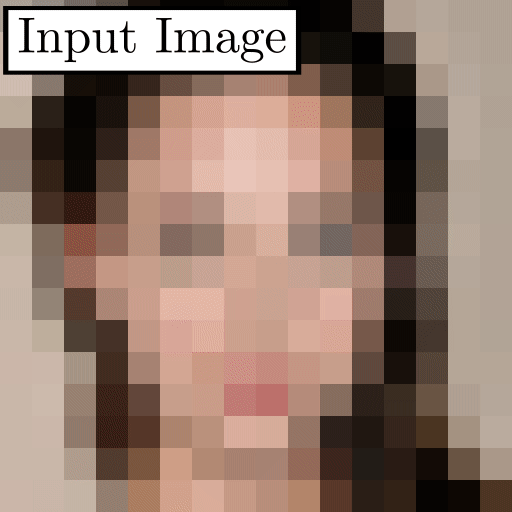
The GFP-GAN model…
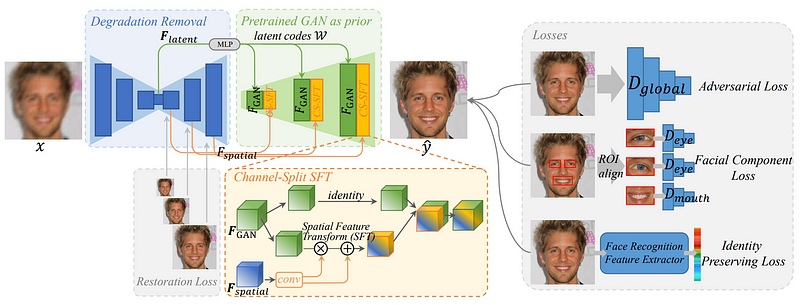
However, as they state in the paper, “these methods [*referring to PULSE*] usually produce images with low fidelity, as the low-dimension latent codes are insufficient to guide the restoration”.
In contrast, GFP-GAN does not *simply* take a pre-trained StyleGAN and re-train it to orient the encoded information for their task as PULSE does.
Instead, GFP-GAN uses a pre-trained StyleGAN-2 model to orient their own generative model at multiple scales during the encoding of the image down to the latent code and up to reconstruction. You can see it in the green zone above where we merge the information from our current model with the pre-trained GAN prior using their channel-split SFT method. You can find more information about how exactly they merge the information from the two models in the paper linked below.
The pre-trained StyleGAN-2 (green section) is our prior knowledge in this case as it already knows how to process the image, but for a different task. Meaning that they will help their image restoration model better match the features at each step by using this prior information from a powerful pre-trained StyleGAN-2 model known to create meaningful encodings and generate accurate pictures. This will help the model achieve realistic results while preserving high fidelity.
So instead of simply orienting the training based on the difference between the generated (fake) image and the expected (real) image using our discriminator model from the GAN network. We will also have two metrics for preserving identity and facial components. These two added metrics, called losses, will help enhance facial details and, as it says, ensure that we keep the person’s identity, or at least we do our best to do so. See the right side of the image above.
The facial component loss is basically the same thing as the discriminator adversarial loss we find in classic GANs but focuses on important local features of the resulting image like eyes and mouth.
The identity preserving loss uses a pre-trained face recognition model to capture the most important facial features and compare them to the real image to see if we still have the same person in the generated image.
And voilà! We get these fantastic image reconstruction results using all this information from the different losses… (watch the video for more results!)

The results shown in the video were all produced using the most recent version of their model, version 1.3. You can see that they openly share the weaknesses of their approach, which is quite cool.
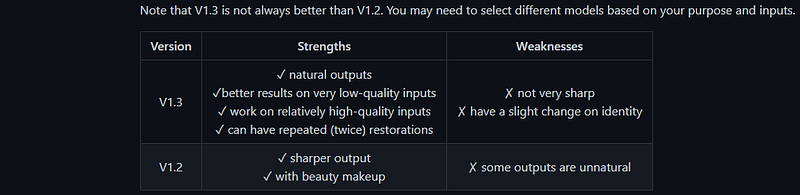
And here I just wanted to come back to something I mentioned before, which is the second weakness: “have a slight change on identity”. Indeed, this will happen, and there’s nothing we can do about it. We can limit this shift, but we can’t be sure the reconstructed picture will be identical to the original one. It is simply impossible. Reconstructing the same person from a low-definition image would mean that we know exactly what the person looked like at the time, which we don’t. We base ourselves on our knowledge of humans and how they typically look to make guesses on the blurry picture and create hundreds of new pixels.
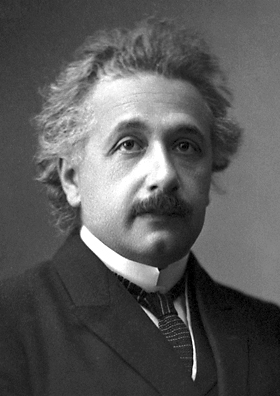
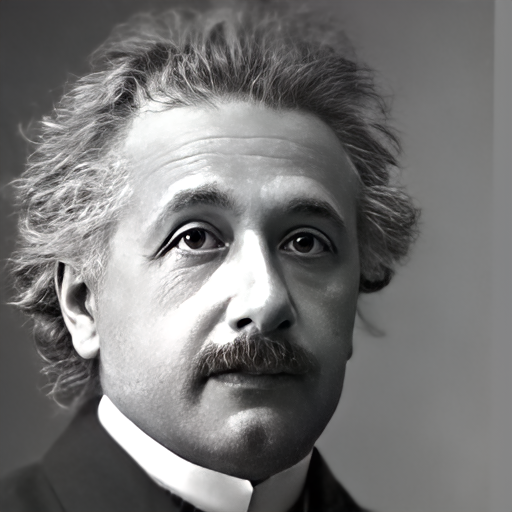
The resulting image will look just like our grandfather if we are lucky enough. But it may as well look like a complete stranger, and you need to keep that in consideration when you use these kinds of models. Still, the results are fantastic and remarkably close to reality. I strongly invite you to play with it and create your own idea of the model and results. The link to their code, demo, and applications are in the references below.
Let me know what you think, and I hope you enjoyed the article!
Before you leave, if you are interested in AI ethics; I will be sending the next iteration of our newsletter with Martina’s view on the ethical considerations of such techniques in the following days. Stay tuned for that!
If you like my work and want to stay up-to-date with AI, you should definitely follow me on my other social media accounts (LinkedIn, Twitter) and subscribe to my weekly AI newsletter!
To support me:
References
- The video: https://youtu.be/nLDVtzcSeqM
- PULSE video: https://youtu.be/cgakyOI9r8M
- Wang, X., Li, Y., Zhang, H. and Shan, Y., 2021. Towards real-world blind face restoration with generative facial prior. In Proceedings of the IEEE/CVF Conference on Computer Vision and Pattern Recognition (pp. 9168–9178), https://arxiv.org/pdf/2101.04061.pdf
- Code: https://github.com/TencentARC/GFPGAN
- Use it: https://huggingface.co/spaces/Xintao/GFPGAN
- Thumbnail image credit: image reconstructed by Neil White (www.restorapic.com)