Third Wave of AI | Thinking Fast and Slow
Drawing inspiration from Human Capabilities Towards a more general and trustworthy AI & 10 Questions for the AI Research Community.
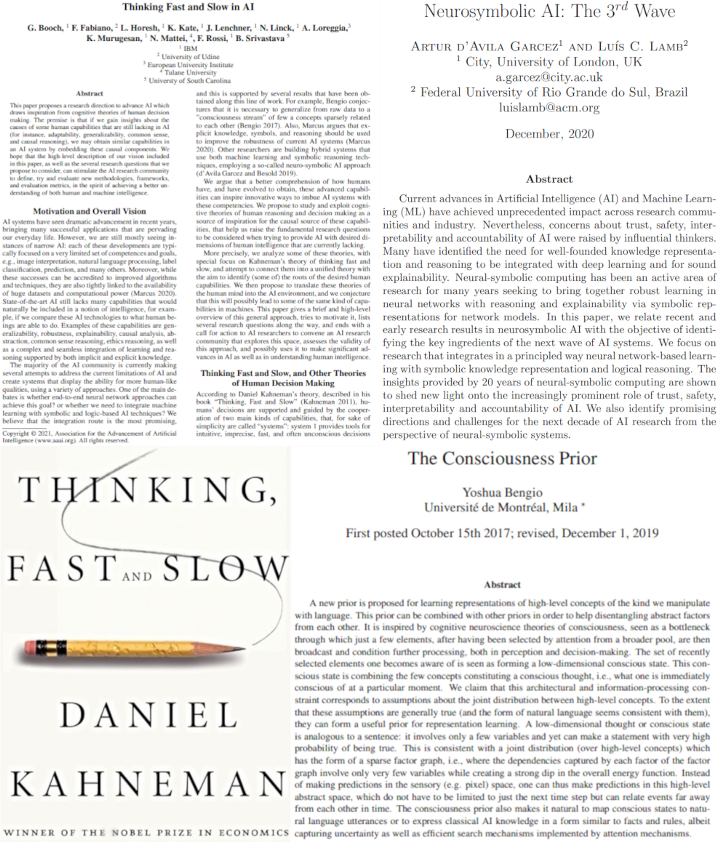
Towards more general and trustworthy AI
I will let Francesca Rossi introduce this article with her great remark made at the AI Debate 2 organized by Montreal AI:
These are the reasons why Francesca Rossi and her team at IBM published this paper proposing a research direction to advance AI. Drawing inspiration from cognitive theories of human decision making. Where the premise is: if we gain insights into human capabilities that are still lacking in AI such as adaptability, robustness, abstraction, generalizability, common sense, and causal reasoning, we may obtain similar capabilities as we have in an AI system.
Neurosymbolic AI: The 3rd Wave
Nobody knows yet what will be the future of AI, will it be neural networks, or do we need to integrate machine learning with symbolic and logic-based AI techniques? The latest is similar to the neuro-symbolic learning systems which integrate two fundamental phenomena of intelligent behavior: reasoning and the ability to learn from experience. They argue that “a better comprehension of how humans have and have evolved to obtain, these advanced capabilities can inspire innovative ways to imbue AI systems with these competencies”. But nobody would be better placed than Luis Lamb himself to explain this learning system shown in his recent paper “Neurosymbolic AI: The 3rd Wave”.
Neurosymbolic is basically another type of AI system that is trying to use a well-founded knowledge representation and reasoning. It integrates neural network-based learning with symbolic knowledge representation and logical reasoning with the goal of creating an AI system that is both interpretable and trustworthy. This is where Francesca Rossi’s work comes into play, with their paper called Thinking fast and slow in AI. As the name suggests, they focus on Daniel Kahneman’s theory regarding the two systems explained in his book Thinking Fast and Slow, “and attempt to connect them into a unified theory with the aim to identify (some of) the roots of the desired human capabilities.”
Thinking Fast and Slow
Here is a quick extract, taken from the AI Debate 2 organized by Montreal AI where Daniel Kahneman himself clearly explained these two systems and their link with artificial intelligence…
In short, Kahneman explains that humans’ decisions are guided by two main kinds of capabilities, or “systems”, which he referred to as system 1, and system 2. The first provides us tools for intuitive, fast, and unconscious decisions, which could be viewed as “thinking fast” while the second system handles complex situations where we need rational thinking and logic to make a decision. Here viewed as “thinking slow”.
Thinking Fast and Slow in AI
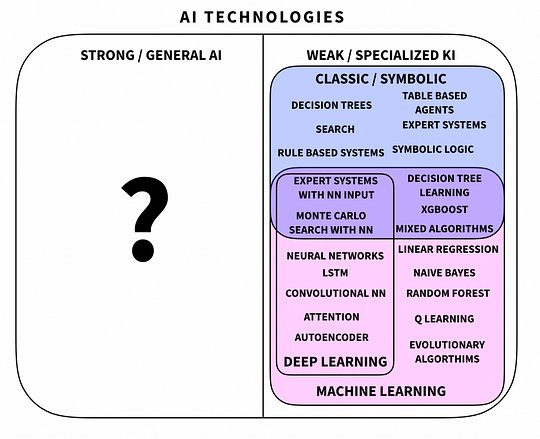
If we come back to the Thinking Fast and Slow in AI paper, Francesca Rossi and her team argue that we can make a very loose comparison between these two systems (1 and 2), and the two main lines of work in AI, which are machine learning and symbolic logic reasoning. Or rather, data-driven vs knowledge-driven AI systems. Where the comparison between Kahneman’s system one and machine learning is that both seem to be able to build models from sensory data, such as seeing and reading. Where both system 1 and machine learning produce possibly imprecise and biased results. Indeed, what we call deep learning is actually not deep enough to be explainable, similarly to system 1. However, the main difference is that current machine learning algorithms lack basic notions of causality and common-sense reasoning compared to our system 1.
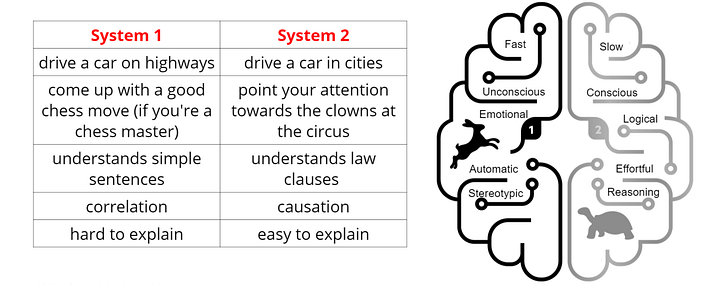
We can also see a comparison between system 2 and AI techniques based on logic, search, optimization, and planning. Techniques that are not using deep learning, rather employing explicit knowledge, symbols, and high-level concepts to make decisions. This is the similarity they highlighted between the humans’ decision-making system and current artificial intelligence systems. I want to remind you that, as they state, the goal of this paper is mainly to “stimulate the AI research community to define, try and evaluate new methodologies, frameworks, and evaluation metrics, in the spirit of achieving a better understanding of both human and machine intelligence”. They intend to do that by asking the AI community to study 10 important questions and try to find appropriate answers or at least think about these questions.
10 Questions for the AI Research Community
Here, I will only list these 10 important questions and contextualization from the IBM paper “Thinking Fast and Slow in AI”. These questions have to be considered in future research with the goal of creating the next generation of AI that could be more general and trustworthy. Feel free to read their paper for much more information regarding these questions and discuss it in the comment.

1 Should we clearly identify the AI system 1 and system 2 capabilities?
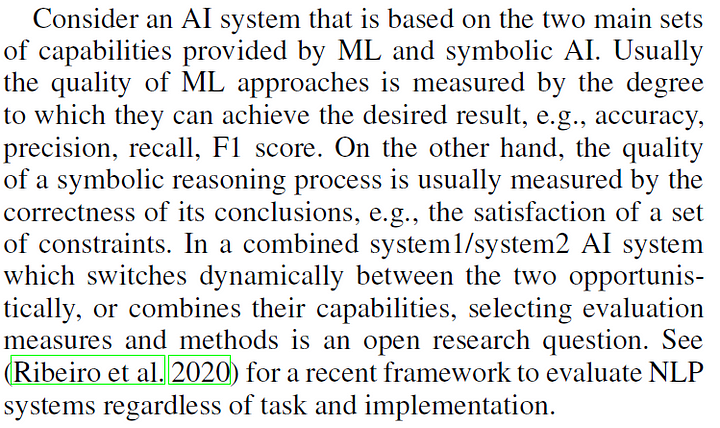
2 Is the sequentiality of system 2 a bug or a feature? Should we carry it over to machines or should we exploit parallel threads performing system 2 reasoning? Would this, together with the greater computing power of machines compared to humans, compensate for the lack of other capabilities in AI?
3 What are the metrics to evaluate the quality of a hybrid system 1/ system 2 (or ML/ symbolic) AI system? Should these metrics be different for different tasks and combination approaches?
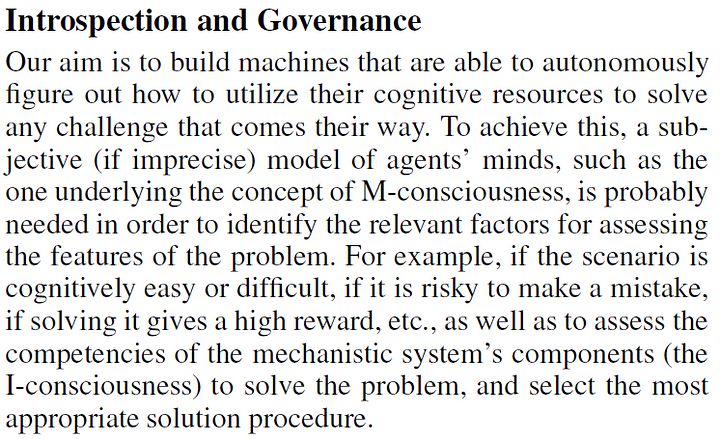
4How do we define AI’s introspection in terms of I-consciousness and M-consciousness?
5- How do we model the governance of system 1 and system 2 in an AI? When do we switch or combine them? Which factors trigger the switch?
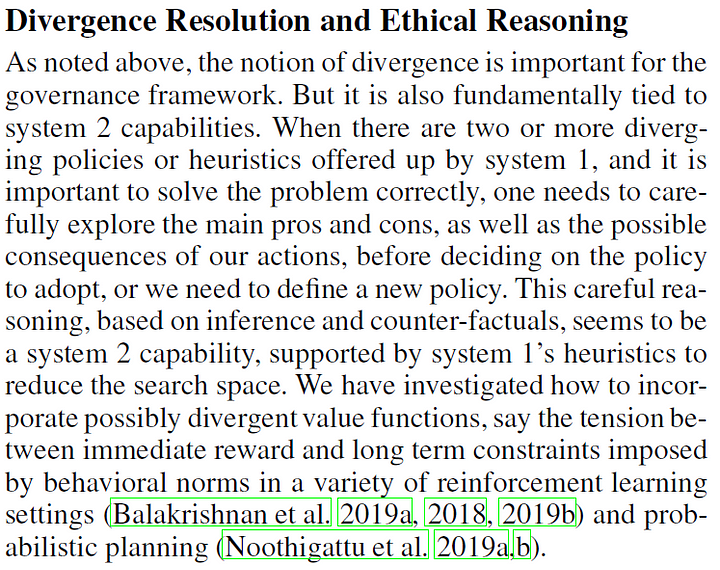
6 How can we leverage a model based on system 1 and system 2 in AI to understand and reason in complex environments when we have competing priorities?
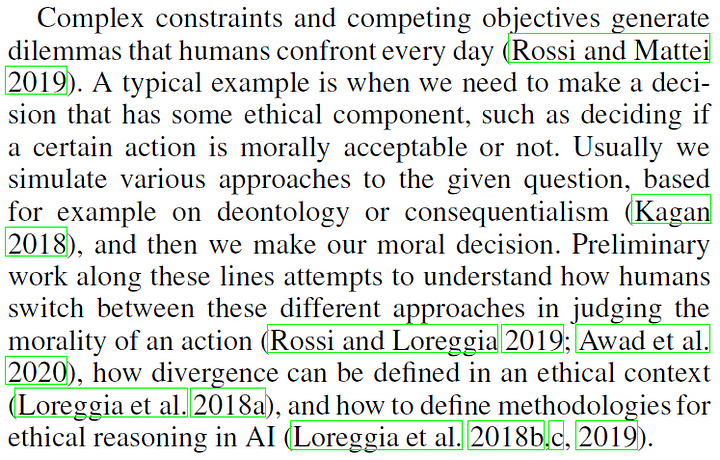
7 Which capabilities are needed to perform various forms of moral judging and decision making? How do we model and deploy possibly conflicting normative ethical theories in AI? Are the various ethics theories tied to either system 1 or system 2?
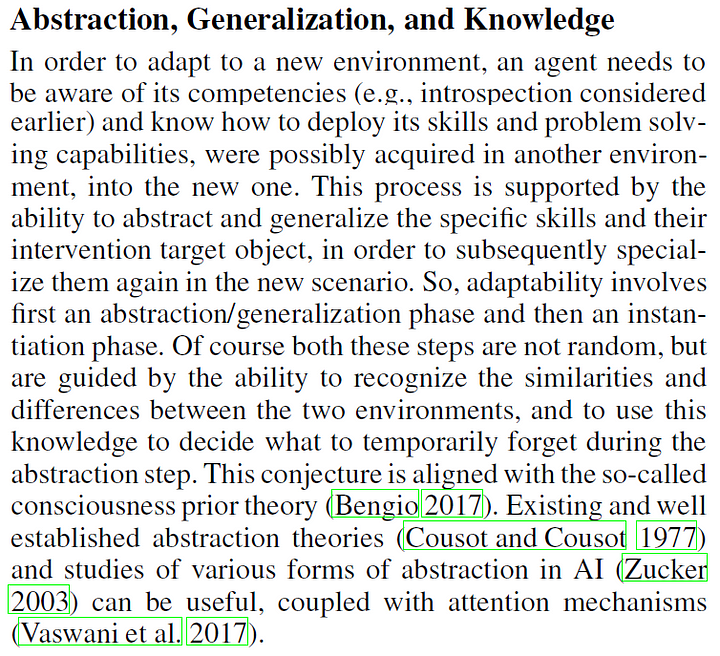
8 How do we know what to forget from the input data during the abstraction step? Should we keep knowledge at various levels of abstraction, or just raw data and fully explicit high-level knowledge?
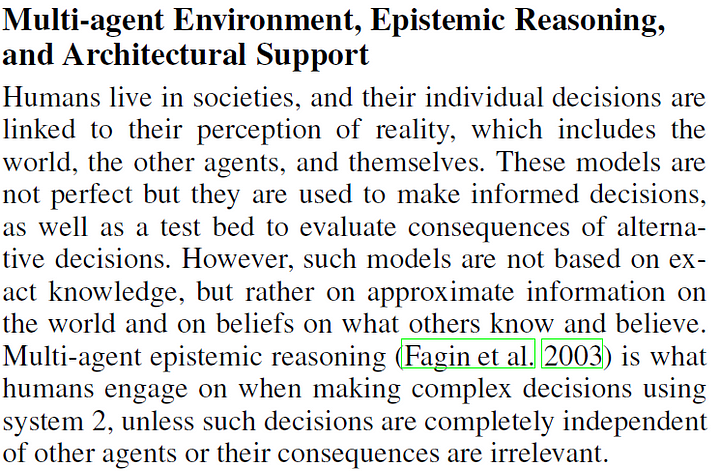
9 In a multi-agent view of several AI systems communicating and learning from each other, how to exploit/adapt current results on epistemic reasoning and planning to build/learn models of the world and of others?
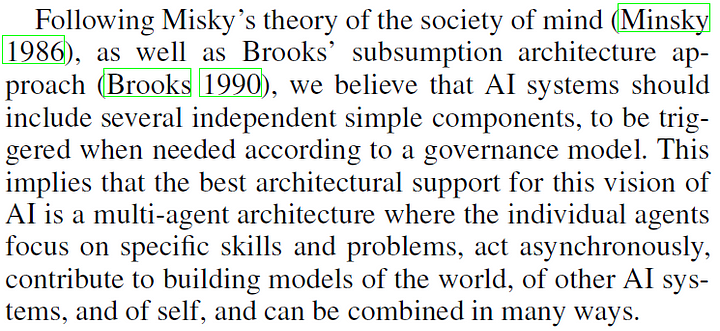
10 And finally, What architectural choices best support the above vision of the future of AI?
Conclusion
Feel free to discuss any of these questions in the comments or message me personally! I would love to have your take on these questions and debate over them. I definitely invite you to read the Thinking fast and slow in AI paper and Daniel Kahneman’s book “Thinking, Fast and Slow” if you’d like to have more information about this theory. If this subject interests you, I would also strongly recommend you to follow the research of Yoshua Bengio addressing consciousnesses priors. And a huge thanks to Montreal AI for organizing this AI Debate 2 providing a lot of valuable information for the AI community! All the documents discussed in this video are linked in the references below.
Watch the full video:
If you like my work and want to stay up-to-date with AI technologies, you should definitely follow me on my social media channels.
- Subscribe to my YouTube channel.
- Follow my projects on LinkedIn and here on Medium.
- Learn AI together, join our Discord community, share your projects, papers, best courses, find Kaggle teammates, and much more!
References
Thinking Fast and Slow by Daniel Kahneman (Book), (2013), https://amzn.to/2XHehG1.
Neurosymbolic AI: The 3rd Wave, Garcez et al., (2020), https://arxiv.org/pdf/2012.05876.pdf.
Thinking Fast And Slow in AI, Booch et al., (2020), https://arxiv.org/abs/2010.06002.
AI Debate 2 by Montreal AI, (2020), https://youtu.be/VOI3Bb3p4GM.