From GPT3 to AGI: Insights from Felix Tao, CEO of Mindverse AI
Navigating the Changing Landscape of AI: Felix Tao's Journey from Researcher to CEO
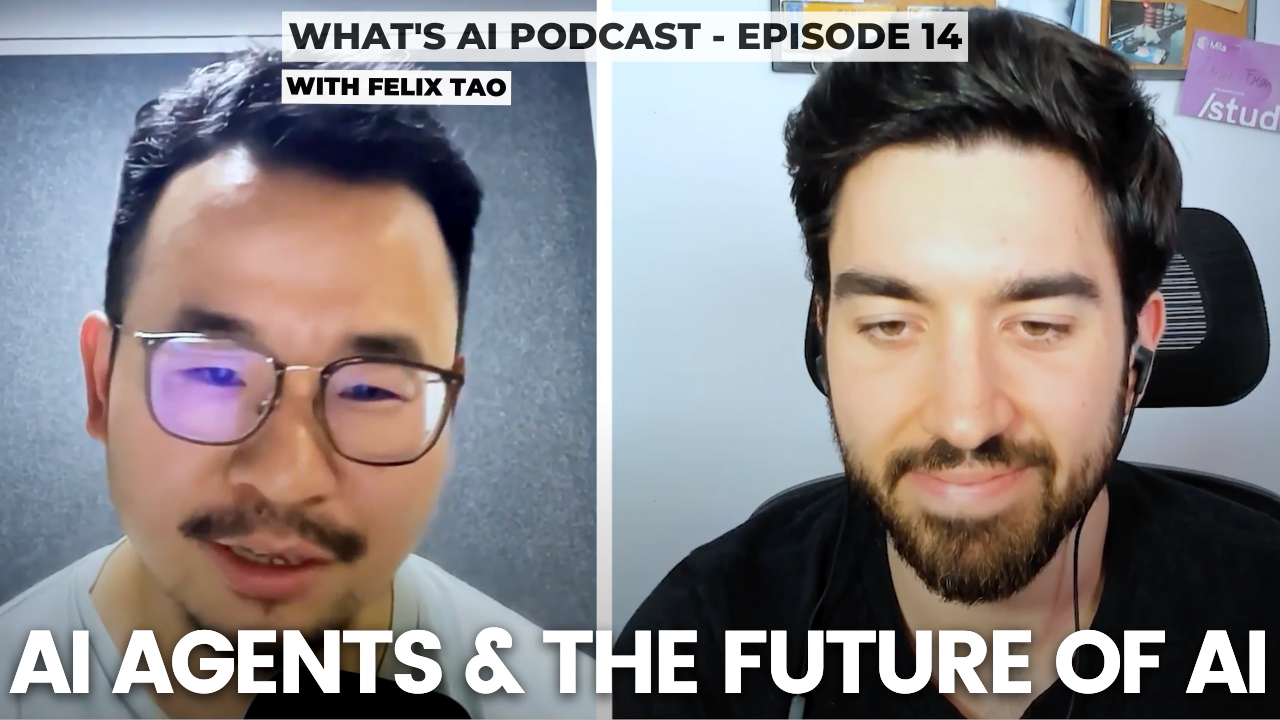
Join us for an episode that's sure to tickle your neural networks as we dive into the captivating world of AI with Felix Tao, the CEO of Mindverse AI. With his extensive experience as a researcher at Facebook and Alibaba, Felix brings a wealth of knowledge in language applications and AI. Get ready for an insightful and entertaining interview where Felix shares his thoughts on the evolution of AI, the marvels of large language models, and the delicate dance between research and application.
As a former member of the Facebook team, Felix reflects on the ever-changing landscape of AI research, with foundation models like GPT3 paving the way for the elusive Artificial General Intelligence (AGI).
But wait, there's more! In this episode, Felix tackles the burning question: Is a research-focused career still worth its bytes in today's world? He'll have you in splits as he cleverly dissects the nuances of the field, revealing the criticality of foundational work while offering pearls of wisdom for job seekers who want to cash in on the AI boom.
Hold on tight as we uncover Felix's time at Alibaba, where he hatched a plan to "wake up the consciousness" in machines by blending large language models with eerily human-like frameworks.
Felix shines a light on the mesmerizing back-and-forth nature of AI development, gracefully gliding between fragmented specialized tasks and unified general approaches. He predicts a future where domain-specific AI reigns supreme, promising higher quality solutions and turbocharged problem-solving abilities.
Don't miss out on this riveting interview that's equal parts informative and hilarious! Felix Tao takes us on a whirlwind tour through the realms of AI research and development and for some mind-bending insights. Tune in now! (or Listen on Spotify, Apple podcasts)👉
Video Transcript
Louis Bouchard: [00:00:00] This is an interview with Felix Tau, c e o of mind verse ai. Felix has done a PhD in computer science before working as a research scientist at Facebook, and then switching to Alibaba to be the director of the Neuro Symbolic Club. Now he's creating his own application, mind verse ai. In the interview we discussed large language models, chat, g p t, mind verse, and a lot of other topics that are really trending right now.
I hope you enjoy it. Awesome. So I will start with my first usual question, which is who are you, and more specifically I will, since it's a very broad question, I, I would like to start with your academic background. So what's your academic background?
Felix Tao: Yeah. My name is Felix Tao. So just like you, I was a PhD student in computer science domain.
So I got my bachelor degree [00:01:00] in Chinua University. And then I came to the states to get my PhD from U I U C, university of Illinois. Especially working on data mining and machine learning stuff. That's the era where before the large language models happen. So, so everything is about training models that can be specialized for solving like particular industrial quest industrial problems.
After I graduate, I went to Facebook in Alibaba as a re as a research scientist. So that's mostly my research background, academic background. And now I'm running a company called Averse, but Metaverse is also like AI related company. So I do a lot of research in Metaverse as well.
Louis Bouchard: That's super interesting. And I'd love to first talk about your PhD just because as you mentioned, I'm also doing one and re retrospectively. Would you say that your PhD was worth it?[00:02:00]
Felix Tao: That's actually, that's a question I ask myself a lot during my PhD times. Honestly in retrospect, I think I think it's worth it for me, but not necessarily worth it for all PhD students.
Because for me it is a very good way, very good training for me to get deep into the foundation of machine learning and AI and truly understand the, and the evolution of how the technology has gone, have gone through. So it prepare me better for, for the future, like research scientist work in Facebook and Alibaba and also in my verse.
But I definitely need to say one thing is the AI industry and AI research aca academia is changing like so fast. So all the research papers I did during my PhD, PhD days are not I, I think they're not useful anymore. So in terms [00:03:00] of impact, in terms of how lasting can your, can, the value of your research works be?
It is pretty challenging to think and for, for students. So I would say if it's, it's, it's, it's worth it as a training process, but not worth it as a way to truly make like hu huge impact on the research area cuz it's changing a lot each year.
Louis Bouchard: Yeah, that definitely makes sense. And I've, I've recently talked with another startup CEO on, on this exact question of research and the PhD, but mostly research, and I don't want to put words in his mouth, but basically the, the gist was that.
Research may not be worth it right now since we've done so much, so much progress recently in research and now it's the time to apply and commercialize and work and, and work on prioritization. Plus, there [00:04:00] are so much opportunities with open AI and just, and minors and everything to help you commercialize and use the models.
So would you think investing in research is still relevant or like trying to pursue a research career path relevant? Or should one go into more like hands-on developer engineering role?
Felix Tao: Mm. Yeah. I think it's a, it's a good question. I feel like The paradigm shift of large language models makes a huge impact on the research community.
One way is the way how we do research is changed, which lowers upon four, lowers a bar for people to, to be able to do research. So previously we need to learn a lot of math and learn a lot, a lot of like tuning on neural networks and patient networks to make something work, which requires a [00:05:00] lot of like study and learning in the related domain.
But nowadays with the help of large language model, we basically trying to do a lot of high level structure on top of that model. So if, I would say if you are not researching on the foundation models themselves it's probably better to skip PhD. And get to the industry to work on things on top of larger language models.
Mm-hmm. And these are the place you can provide as a maximum value. But if you are researching on the fundamental math and mechanism of larger language models, or we say foundation models, then yes, research is still very valuable. But that also means like previously we have like CV area, NLP area, and in LP we have so many different tasks, right?
All these tasks, many of them are gone. It's not a valid research problem anymore because they can all be done by a [00:06:00] single foundation model. So to study these models, to study these questions is probably not good. Not valid anymore. Yeah.
Louis Bouchard: Yeah. It must be difficult compared to back in the days to find a research topic, especially in the PhD or for students like.
Relatively beginners it, it must be difficult to find a research topic that where you can be impactful versus open ai, deep mind meta and every, every large company in the field. It Yeah, it's definitely not only that, the PhD itself takes a lot of time and may not be as valuable to yourself in terms of like income.
Mm-hmm. Compared to having a job and even to the learning process. Like working in a startup or having your own startup is like, you learn a lot, you are super motivated and you work way more just because you wanted to succeed and you, you, you learn a lot just [00:07:00] like in the PhD. Mm-hmm. But I don't know what will happen with the PhD.
Like, I don't know if it'll stay the way it is. I see more and more work being done affiliated with companies. So I assume this is where it'll go, like most PhDs will be people doing a PhD format, meta, for example, and things like
Felix Tao: that. Yeah, yeah. Sadly, sadly, I, I agree with most of, of your points be because the, the resource requirements to, to get something down on AI space the, the research labs from universities are not gonna be as suitable as industrial labs, right.
For example, the, the, the AI labs in Google, or in fair or in, in open ai. So, yeah. Yeah. I, I think, I think this. This kind of shift happens to many many industries. For [00:08:00] example like database research, mostly in the early days, all these innovations are done by research labs. But as after the database systems becomes more commercialized and the commercial companies have have more resource to, to pull into the research area and building their own like product, than many innovations are done by the industrial labs or done by the commercial companies.
I think AI is having this kind of moment. So after that the, the field is still gonna be very innovative, innovation driven, but I think the majority of innovation would come from the re from the industrial labs or startups like, oh yeah.
Louis Bouchard: Yeah, I definitely think so as well. And before diving into the topic, you mentioned that you worked at Facebook and Alibaba, so I would love to hear a bit more about what were you doing first at Facebook?
Felix Tao: My, my work in Facebook is [00:09:00] very interesting cuz Facebook mostly is, is is one of the biggest platform in the world to have like so many data, like so much data including like documents, news, articles, videos, pictures. So when I joined Facebook, Facebook is having a new direction. They, previously you can only see like friends post in your newsfeed, right?
But they think it's people are getting having a lot of demand on truly using Facebook as an information source to get like news updates and to, to get interesting videos, not just from friends. Status. So at that time they, they're thinking about how can we bring bridge the users billions of users on Facebook to like the billions of piece of informations on the web.
So trying to bring information to people. And then we needed to develop a tool, we call it content understanding [00:10:00] tool. Basically it is a AI platform that can, you can put all the information, all the news, articles, images, videos into this platform, and it will be able to understand the topic, understand the key concepts embedded in those content.
And Facebook can use these kind of signals extracted by AI from this like text data or video data to help. Users to find those relevant informations based on their interests. And I was a person who started this project in newsfeed. They're trying to understand text data first, and then go from text data to image and video data and trying to build a good foundation for AI foundation for content understanding.
And that was actually my first job and it's really exciting to, to, to be able to work on a project like this of [00:11:00] this size. Cause you are basically handling like the world's biggest database. And I try to use AI to, to help understand it. So that's very cool.
Louis Bouchard: And just to put that into perspective, when was this exactly like what year?
Felix Tao: I started working at Facebook from 2017.
Louis Bouchard: Well for, for ai that's like a, a very long time ago compared to where we are at now.
Felix Tao: Yeah. I, I think at that time we, we don't have the concept of large language models. So the way how we understand those text data or video data is still quite traditional in AI sense.
We, we develop like specialized models and to extract topics or find the, like people in the videos or these kind of things. They are done by a set of different models. But nowadays you, you, after the help of these foundation models you probably can do them with a more elegant way [00:12:00] by using one single model to handle the whole content understanding job.
Yeah. It
Louis Bouchard: must have been a very good role to, to learn a lot about natural language and all the, well natural language processing, but like all the, the intricacies with understanding text. Mm-hmm. Yeah. Like, since you didn't have all the tools that we now have, which makes it much simpler, even though it's extremely complex, but it's still like the, well, the challenges are very different, I assume, as well.
Felix Tao: Yeah. At that time the NLP tools we are using, it requires a lot of like, you know, data labeling kind of stuff. And which particular task, for example, like topic extraction from news articles we need to label a lot of data. So that's why in Facebook or in any tech company invest heavy ai, they usually partner with all these like data [00:13:00] labeling generation companies.
Where they hire people to label data for their tasks. But nowadays, as you say, all these tasks can just be a prompt to, to, to be a, you need to design very smart prompts for the large language models. And all the tasks they do is to how, how can we train a large language model that can observe all the data on the web and to, to let the model learn how to understand the whole world.
And it's, it's a totally different topic.
Louis Bouchard: Yes. Really. Cool. And speaking of different topic, it seems, I, I've read that you've been the founding director of the Neuro Symbolic Lab in Alibaba. And so I, I've also read that the goal was to. Waking up of consciousness. And so this seems super different from what you are doing at, at Facebook prior to that, and I, [00:14:00] I'd love to hear more about it.
Felix Tao: Yeah. I was going through a very interesting time in AI history. When I started, I once started to quit my Facebook job to John Alibaba. That was around 2019 and and 2020. So around that time, there is a very big thing happening in AI industry. We all know is GP three, right? So Open Air launched the GP three around the middle, mid of 2020.
And around that time, I feel like how we approach ai. It's done wrong because so the mindset sets all these AI researchers have before is always how can we define the problem to a particular model, and how can we define the inputs and outputs of this model? Yeah. And how can we get data? And no matter it's by labeling or by harnessing from the, the a, the web, how can we get data, enough [00:15:00] data for this particular task?
But after GT three, I realized that the, the goal of AGI has a very good foundation, very good starting point scientist in, in the early last century. When they define the term ai, they mean agi, that AI can learn things and can do all different kinds of jobs. But because that, that is too hard at that time, that's why people start to Have different directions.
Like some people research on the vision problem. How can machines see some people research on the NLP problems, how can machines read and understand the text? And then you go deeper and deeper. You, you, you have a set of problems in nlp and for each problem, you split it into smaller problems. That's the, the traditional mindset for AI researchers.
But with after g PT three comes out, everything's [00:16:00] changed. So that, that's a time I realized that, that maybe we can do something different. Maybe we don't need to follow the traditional way of doing AI research. So I think that's why I started at this research lab called Neuros Symbolic Lab.
So I tried to. Combine foundation models, large language models together with a framework which is more like human brain, for example. The, the framework is able to have like truly long-term memories as the framework is truly able to have like perceptions getting all these different kind of information sources and put it make them useful.
And you just send these signals to the foundation models and let the foundation model to process all these signals for you. So if we combine foundation models, f combine large language models with all the memories, perceptions, and even like abilities to model control to be able to [00:17:00] control the, the arms of robotics, those kind of things you are actually making something similar to a human.
Right? Yeah. So you are ma making some, some you are truly pushing AI to be like how a human process information, how a human think about a problem, how a human deliver its actions. So I feel like it's time to do that. So that's why we set up a very ambitious goal, saying like, waking up of the consciousness in machine.
But it's probably not done yet, even from today's perspective. It's, but I think it's much, much more closer than five years ago to, to truly achieve this like ambitious goal.
Louis Bouchard: Definitely. Just like with, well, I don't know if it's recent in term of AI history, but with Clip we've seen that we could understand a concept.
From either text or images, [00:18:00] which is also something that we can do quite easily if we see the word cat or a cat. Mm-hmm. Or if we hear cat, we can all link it to the same concept in our brain. So that's pretty cool that right now we can do that with AI or with one model. Yeah. Well one larger model. So that's, yeah, it's, it's really cool that we seem to be transitioning into foundation model, basically, but Yeah, into larger and more general intelligence compared to being very good at a specific application.
Where we were aiming at, we, we definitely were aiming at that just a few years ago, even in my master's, was to do some few shots classification, like on, on ver be better on very specific task, and that mm-hmm. That's, mm-hmm. Already much different now, so it's, yeah. Pretty
Felix Tao: cool. Yeah. And one, one thing I I, I'd like to add is I, I also observe the dev evolution of [00:19:00] ai.
And I think one pattern is very interesting is we start this AI concept, AI research field by having a bunch of like super intelligence researchers trying to tackle the general AI problem and then failed first. And then we start to take this fragmented approach where we split the tasks into smaller ones.
And by having like hundreds of different AI tasks and having separate people working on each of them then it goes back to a more like unified. Approach you more like general approach. And I don't know. I, I, I feel like then after this having, having these large language models, then we probably will enter into a new era where we find one large language model is not gonna be sufficient enough.
We want to diverge from it again, to have different types of large language models for different, for example, personalities[00:20:00] for different like jobs. So it's always like fragmented and then unified and then fragmented. So, so this is a very interesting pattern happening in ai. That's why I think, as you said, even though nowadays we see only a few companies are able to deliver the, the top.
Large, large models, but I still think in the future no matter it's startups or maybe research students, they would be able to find the niche issue, niche, niche problems to solve. But let's see. Yeah.
Louis Bouchard: And regarding spliting into smaller applications, would you think, wouldn't you think that's that's already what we are doing with, for example, lots of people take large language models and fine tune them to their own task or mm-hmm.
Rather, sometimes build a memory of some kind of data set that they have, for example. Mm-hmm. A medical book and then they just ask the large language model to, [00:21:00] to cite this medical book. And so we already are, are doing that, like going from the large model and, and. Adapting into our, adapting it to be better on, on our specific task.
Yeah. So do you think this is promising avenue or, or will we have a large language models soon enough that is better at each of these very specific task versus splitting
Felix Tao: it? Yeah, I, I think that's a very interesting question. So to be honest, I, I don't have a certain answer yet, but my, my belief is I feel like we need different AI for different tasks and just to make it more specialized and making more more of high quality in terms of solving that particular domains problems.
For example, my company is called Averse, right? So we have this core product called Mine os It's basically [00:22:00] trying to solve these four different domains. Experts. I, I can I I would say that large language models has two things embedded in the model. One is their reasoning ability. So they are able to reason by com complicated logic, no matter, it's, it's domain agnostic, so it's not related to some particular domain.
It's just how they, like human beings. They can just use logic to reason use logic to solve problems. And another layer of large language model is their common sense. So this common sense of the understanding of the whole world is obtain when they, in the pre-training stage, when they go through all the web data.
But this common sense usually are not good enough for a particular domain. So that's why people can particular domain, they probably need the reasoning part, but not necessary, the not knowledge part embedded in larger language model, [00:23:00] because they want to have their own specialized knowledge. They want to have their own vertical data.
So we call that the grounding layer, right? So we use large language models for reasoning, but we add a grounding layer on top of it to make the model tuned to a particular domain. So they will be much, much better solving at that domains problem. I, I think that's that's one of the major goals for mine os so people can do it in a very easy manner.
They can simply updating documents and they can simply connecting domain specific abilities. APIs. Onto the model and the model itself would be able to plan and retrieve related information from this particular domain, particular workflow, particular use case, and use the reasoning power of larger value models to, to solve, its better.
It's basically
Louis Bouchard: like replicating the process of [00:24:00] a perfect human where we, we are all able to eat, walk, and do pretty much everything, but yeah, you have to go to university and do a PhD and everything if you want to be the best at something. And so it's funny how we are basically trying to do the same thing with ai where we try to teach it the world in general ju just like we do to our children.
And then we, we give it like lots of very specific documents, just like we do with university students to become experts in one field. Yeah. So that's it. It completely makes sense. Yeah.
Felix Tao: Yeah. To totally. I, I think the way how you put it is a per perfect way to describe how we build these AI systems. So, so you need like all these pre-training steps, like training a kids from just born, born to having like a college degree.
Then you need this professional training to make it like [00:25:00] experts. In a particular domain. So what we do inverse, what Open AI has done or many other large learning model has done, is to finish the pre-training to make sure the AI is like a college grad, graduate. And but for, for what we do is to have this like professional training agenda designer for each ai so they can be super professional in their domain and they can have long-term memories.
So they cannot only be profe. They, they're not only professional, they can also grow as you use it. So they can grow more and more grounded into your particular domain and your particular use case. So that's very exciting because for me, in the future, I think AI is not gonna be a general model solving everything for everyone.
Each person, each business, they, they. They need the AI to be more related to them, to their [00:26:00] life, to be more grounded in their daily work. So adding this grounding layer on top of the large language model is definitely something that can have a lot of innovation happening. So
Louis Bouchard: I assume that is the main goal of mine os is to build these additional layers above the, the first initial pre-training.
And are you aiming to do that on most fields or are you specializing to ver to some, some fields. What exactly are you building and aiming for with minors?
Felix Tao: Yeah. Yeah. So metaverse, the, it it, it's like our vision, right? So it's the term metaverse basically is our vision. Where in the future AI beings are gonna.
Coexist with human beings in a form, a new society where a lot of things humans do, human do are gonna be delegated to ai. And [00:27:00] AI is just gonna be integral part of our society. That's a vision. I, I think we can try to make it like a bene a good vision, a good future for, for humanity.
And mine os is our product. So basically it's like a operating system that can generate AI mind and we call it AI geniuses in, in our system so anyone can get on the system and trying to create a AI genius for a particular domain. For example, you can create a AI genius for, for like a hotel bachelor.
You can create a AI genius for your, like, assistant for AI research, right? And genius for HR support, all these kind of things. So, To do that, we, we need to, like you said, we need to tackle a, a couple of technical challenges. One is to make them like easy to use and add this grounding layer on top of each AI genius.
So we are [00:28:00] making it as general as possible to, just to answer your question, because the, the foundation model itself is general. Yeah, right? And the, the training process, the professional training process is mostly alike in the real world. So what do we do? The grounding layer is basically adding the training procedure for different domains.
The, the, the way how you train it is sim similar, but the, the, the material, the, the data you train it is different for different domains. So mine os is mostly trying to provide a tool set. Engine, a platform that different domains can use. So we don't try to focus only one or two domains and we want to make it more like a create creativity platform where people can have their own creative way of generating AI geniuses for, or AI minds for their own domain.
So [00:29:00] that's a goal, that's one of the biggest features in mine os. But we also do other things to name one, which I think is very interesting as well, is like when you use chat G B T, you know the reasoning power is there, but it can only do one round of reasoning right after you ask something, it gives you some result after reasoning.
But we can already see a trend in industry, for example, auto, G B T, so the AI would be able to autonomously use its reasoning power, and By different, by many iterations. So it's like you are multiplying the reasoning power of large language models by a big number for a particular task. So they would be able to simplify complex task to different sub-tasks and do it gradually iteratively.
So I think that's very, also very important piece of work we do in mine os to let AI, to have the ability to deep think, [00:30:00] to do like slow think so they can leverage the power of reasoning more and make the AI more powerful.
Louis Bouchard: Yeah. I have lots of questions following that, but. With the last thing you said.
I personally using C G P T and other models, my main issue is hallucination. Mm-hmm. And so I'm a bit afraid and, and skeptical when talking about chaining those prompts and requests just because I feel like it can just grow the, the risk of hallucination and just working up on these hallucinations and just like growing more and more the what, like the, the wrong it can do.
And so is there anything you are doing to mitigate the, the risk of, of hallucination or is that a thing that the brands using mine os need to tackle? Is that, like, is there something you guys do to, to [00:31:00] help with that?
Felix Tao: I think hallucination is, One of the major reasons why people, especially businesses, don't use chat G B T directly.
Yeah. And to me, I think the solution to this can be twofold, right? One is how, what Open AI is doing. For example, they're training TBT four and maybe even like higher level of logic launch models in the future. I think one major goal of these new, new models are to solve the hallucination issue.
So I think in one of the interviews by done by Sam Altman, or Ilia, I can remember whom they say that the hallucination issue in GB four is reduced by, it's at least 60%. So, so that's one, one area where people where we can make it better. Another area is what we are doing is the, the grounding layer.
So by doing grounding layer, we use like tactics, for example generating like very special prompt to [00:32:00] enforce the AI model to speak on things by the reference text. Yeah. Not by things trained from the pre-training stage. And we enforce that. We also added this like we say citation system.
So everyone say, he said we would like to ask him to add citations from the original source. And ev everything marked with the citation would be more trustworthy than things that's not marked by citations. So it can solve some issues when people perceive the result of the AI generated. Right. We, we can have more trust on things they, they think have a good citation on and things they don't have a good citation on.
But I would say the, the hallucination issue is a, is a fundamental flaw for large line models. And I, I actually think it's a fundamental flaw [00:33:00] in human mind as well. Yeah, yeah. So, so humans sometimes do a lot of like bullshitting as well, so yeah. But I think it's, it's getting solved gradually.
In, in areas like marketing and for example entertainment people are more like okay to have these hallucinations sometimes as, as amount of hallucinations controlled. But in areas like medical, as you say, medical law, and all these kind of very serious domains, probably this is gonna be a bigger issue.
So I see even different industries when they adopt this large language model, large language models, they, they have like different pacing for adoption. Yeah.
Louis Bouchard: And that's actually how I used to describe it as well, large English models. I just, I, I used to compare it with Wikipedia where mm-hmm. Like back in the day, you, you can't trust Wikipedia, but you cannot really cite it or you cannot [00:34:00] write a.
Something for school or whatever. Mm-hmm. Based on Wikipedia, you need to check the the sources and Yeah. Confirm. So it's pretty much the same thing with large language models right now. You, you always need to confirm what it says if it cannot cite where it took its information. Which is why I think like linking it to a memory based on mm-hmm.
On lots of documentation is, is super powerful and maybe the easiest solution to, to tackle that. But I agree that it may be just like a human bias that is just generalized to language models, just because lots of people share fake, fake news or, or lie to their friends and stuff like that. And the data is, is, is from us.
So Yeah. Definitely makes sense that it's doing the same
Felix Tao: thing. Yeah. Yeah. Totally. Totally. That's a very good analogy. By the way, I think ju just like you said, one thing we can always do to reduce the impact of hallucination is trying to make the thinking [00:35:00] process of AI as transparent as possible.
Yeah. So for example in the stage of retrieving informations as external reference, we make it transparent in the process of calling a APIs to finish a task, we make it transparent. So the user, once they talk to a AI chat bot with powerful chat bots, all the actions, all the information used in the whole thinking process would be, should be transparent so people can have more trust.
And this, this cannot be done by a real person, right. We cannot, when we talk to a professional, we cannot ask them to, to list all the thinking process for us. But we can do it with ai. So, I think we have different a lot of, a lot of ways to, to reduce the impact of hallucination. Yeah. Mm-hmm. Like that
Louis Bouchard: I just mentioned that it's important to double check what the language model says, just to be sure it's, it's truthful.
And so when it's chaining prompts and [00:36:00] you are not controlling everything is there a way to ensure that what is happening in between the, the input and the final results is, is truthful and there were no hallucination in between?
Felix Tao: I, I don't think we can, can't, nowadays we, we don't have the proper tools to get into the, like, very detail of the computing process done by large language models, right?
Yeah. Because it's more like a black box. But I think to train a powerful ai, you probably don't simply just use large language models, you actually build a framework on top of it. And having these, like thinking thought flows between different parts of the mind you have thought flows from the memory to the perception area, from perception area to the you know, motor action area.
And this high level flow of information can totally be transparent [00:37:00] to the users. So I'm not sure if open is, it, is developing something, trying to visualize and make the, the hidden process transparent. I doubt it's very difficult to do, but we make the high, high level process transparent and we make sure everything the, the, the AI generat generated to user have some good reference and good citations.
I think that's, that's one way to go, but it's not gonna solve the problem by 100%. And could
Louis Bouchard: you identify what is right now the biggest challenge when build, when trying to build these, well, this tool that produces specialized agent? Like is there one specific challenge that is something you are currently working on and is harder to solve than, than the others?
Felix Tao: One thing is just as you said, hallucination. But other than hallucination one, one big [00:38:00] challenge that we try to tackle is how we actually make the AI as grounded as possible. Usually people's knowledge on how to deliver a job is very complicated. It's not only about. Providing AI a few documents.
It's not only about providing AI with a couple of tools. You, you always need to teach the AI how to behave in very different scenarios. So we can definitely do it by give instructions to AI in different scenarios. But still they sometimes don't understand like the best, best practice for a particular domain.
They have these different tools, right? For example, if we are building a marketing agent, they have tools to connect to Facebook. They have tools to, to finding the product details of a company. But how to combine them together for a better marketing campaign campaign [00:39:00] is quite challenging. Cause you can use some piece of information to give, like, give us some good ideas, but for them to autonomously finish it for you.
Is very hard. So g B T and similar like technologies like what we are doing can be a good direction to go, but this autonomy has issues. For example, they are not controllable. They usually can be very open-ended and not very focused on the particular task. And which turns out to be like wasting a lot of money, but not solving your issue.
So how can we make AI that knows how to deliver a complicated domain specific task? And without random thinking about like, random ideas, that's very hard to do. So I would say no matter it's a hallucination [00:40:00] issue or the like, Autonomy issue. It's all about how we can control the AI's behavior to what we really want it to be.
By natural language. That's a very key part. That's why we need to build a really, really good framework on top of large language models to deliver the know-how to deliver some feedback to the AI so it can smartly incorporate users' feedback into the way how they think and the way how they perform tasks.
Hmm. That's very hard and that's a major technical challenge we are facing and we try to solve it by, by the framework we developed.
Louis Bouchard: And is the, the only way to tackle this is through, is through prompting and trying to like, Plus training, make it understand how to do the job? Or are you also referring to fine-tuning and [00:41:00] basically changing, also changing the brain of the ai just to, to tweak its answers.
Like is it fully after training with, with prompting resources or also related to retraining or fine tuning the models?
Felix Tao: Yeah, it's, it's also a very good point. I think currently most people are doing, using this prompting approach. The way how we do it is not ask a human to write better prompt.
It's like how can the AI get some feedback and updates their own prompt, basically update updates their own behavior by updating their own instructions. So that's one way automatically. And if, if it can be done automatically, it would be a very efficient way to tune and control AI behavior. But I think this approach has some limitation and it cannot achieve like, total control over AI behavior.
So I, I believe in the [00:42:00] future we probably want to have some training built in the process. So we don't train the, the foundation model. We don't train the big one, but we can definitely train a behavior module on top of that, which is, which can be a very small model like adapter. Yeah. And this model would, would be in charge of getting users feedback and updates the parameters within it automatically and gradually adapting to user's preference And Make it more grounded, more suitable to what a one user really need.
So, but the, the prompting approach is gonna last for a while before the real fine-tuning stage comes because we haven't seen the, we haven't reached as a limitation of the prompting approach yet. So it's always more convenient to work on the prompting part. Yeah,
Louis Bouchard: yeah, that definitely makes sense.
Mm-hmm. And I, I had [00:43:00] a, a question for, this is mainly for a friend of, of mine that is it's about mine, verse and mines, but it's, it's also to give context and, and help the listeners to, to better understand the tool. So I will just yeah, give my example. So my friend is a recruiter at a company, so, and he tries to find people to fill in a broad range of roles.
Mm-hmm. And right now he tries to find ways to use AI to improve his work. So, okay. He doesn't have any background, like he's not a programmer. He's not, he doesn't have any background in AI other than playing with Judge G p T. Mm-hmm. And so how could someone like him, which I assume a lot of listeners are in the same profile, could, could use mine os or mind verse to improve anything in their work, and like, what do they need to learn or to do?
What would be the, the [00:44:00] steps to get into it and have an agent help them at in the end?
Felix Tao: Yeah. So, so if I understand correctly, your friend is in the HR space, right? Yeah. Okay. So definitely I think I, I believe in the future, any professional like hrs, like lawyers and researchers, they can, they can have their own way of using mine os or any other agent platform to build their own agents or just use other people's agents.
For, for their work. For example, if we look at this HR job, you can find many different steps, right? Some steps are trying to find the new candidates from the web Yeah. Or from some like linking profiles. And then the next step is trying to assess the degree of feeding of each candidate to the job description.
And then maybe we can have this like interview process and then communicate with the candidates or this kind of compensation or these kind of things. [00:45:00] For many different things, you can definitely use minors to build one agent for your job. So for example we in minors for example, you, you, you, when you try to find a good candidates from LinkedIn, we can add one endpoint for the genius you create to have the ability to, to browse the web, especially on the LinkedIn websites.
And then you can create a very good workflow that by dragging a few modules together, say any candidates you find, you first assess them based on their for example past experience and give them a score from one to five. And this second thing is mo mostly by issuing a natural language command to the ai and ask the AI to do it as you order it.
To do so, they will start to automatically browsing the web and getting all these linking profiles and trying to[00:46:00] like grade them by their experience. And then you can build another workflow on top of that. Say after you grade them, please send all the like Five grade as five candidates to my email and rank them by the closeness of them, their current job to our city, something like that.
So, so it's very, very easy to set up those kind of workflows in monos. And we, we have all this, we, we actually have a very interesting feature in Monos. We are contributing is we are creating this collaboration network between AI agents or we call AI geniuses. For example, you, you, you create one AI agent for your like talent acquisition process.
And you have another AI agent working on this like resume grading. And I have a third agent working on this like automatic [00:47:00] interview with with candidates. Per se. So you can ask, you can connect them together and say I need this ai like talent acquisition agent to first find the talents and then pass this to the second agents for grading and finding the fitness, finding the fitting positions in within our company.
And then the third one to start an initial interview with with with candidates. So this, this kind of way to work can be applied to all different types of jobs, right? Not only for hr. So we can always find this like each job they have different components and each one requires them to teach the AI how to do it properly.
And if we can have this mechanism to combine these different AI together we can largely reduce how people work on this, like repetitive and tedious work. And that's ma mostly the goal of mine os and that's, I think, [00:48:00] answers your question, how can they use mine os and to improve their productivity.
Yeah.
Louis Bouchard: Yeah. So just to summarize, it would almost only be through lateral language, so English or even I assume it could work in French or other languages. Yeah. Yeah. And do they need any other skills? For example, when you say that the, the agent could go on LinkedIn and, and scrape all the, the different profiles, do you need to, to do anything related to connecting it to LinkedIn?
Felix Tao: Yeah. We already provide a, a set of like public skills, for example, ability to browse the web, or ability to run some code, ability to, to find a flight. For your travel things like that. But the, the beauty of it, the beauty of the grounding layer is for your particular work, you can always have your own vertical abilities, right?
For example, you can, you, you need to [00:49:00] connect to a vertical database to look for some information or, yeah. Finding out the, the, the clients from your cm, what, whatever. You can always connect these vertical skills vertical tools into the ai. So they, the AI itself would, would be a, be able to autonomously.
Use this set of skills and trying to, for each of your inquiry each of your tasks, they would be able to automatically come up with a plan using these different tools and solve your particular issue. If you think it's not good enough, add more tools to it and give more feedbacks to the ai.
And you have become more powerful and trying to adapt to your true need. And I think that's the power of minor wise. So, Like, like I said, it's a, it's a [00:50:00] tool. It's a very good tool and you can build use this tool and for your need, and we provide a lot of flexibility to users so they can basically build anything they want.
Louis Bouchard: Really. Cool. Yeah, it's, it's really cool and I'm excited to see what people will do, not requiring coding skills or learning other things to use those models. It's, it'll just, I think it'll, like the quantity of user will, will speed up the development progress as well, just based on all the investments and mm-hmm.
What it'll s simulate with that. And so I have a few final questions just related to the, mainly to the future, but mm-hmm. Yeah, it's, it'll be difficult questions, but short ones. The, the first one is, is there a thing that AI cannot do yet, but you would love it? To be able to
Felix Tao: do. I think the AI countries still don't have like some [00:51:00] self-consciousness, but, but I, I'm not saying self-consciousness as a very risky way.
It's more like AI is self-aware of what they can do and they cannot do. That's very important. That's, I think that's a major source of hallucination. If they know that they don't have sufficient knowledge or sufficient capability to finish something, they should be able to realize they're lacking of this kind of capability.
And then they will stop being halluc, generating hallucination generating like false informations. So I think it's very important to build some sort of like self-awareness module in the AI agent or in the AI mind framework for them to not only to understand what they cannot do, but also understand that they need to learn new stuff.
To grow themself, to be self-teaching. And that would some be something [00:52:00] super helpful and super cool. And I don't think, I don't see any AI tools or air frameworks based on large, large language models, can, can have that down.
Louis Bouchard: I completely agree. And self-teaching will be super important, but I, I, I'm afraid it's also something that humans are good to.
Just the typical fake it till you make it is basically exactly that. Some humans assume they can do something or just Yeah. Fake that they can do something and they, they cannot. And so AI is just doing the same thing. Yeah, it's true. It's true. And so, okay, I ask you about the biggest challenge facing your, your company right now.
But. Would you have a different answer for the biggest challenge facing the whole AI industry in general? Or is that the, the main priorities for, for most people you
Felix Tao: would assume? [00:53:00] I think about that a lot recently, especially after seeing the power of TB four. So I think the biggest challenge is how can we control the impact on the society.
As a whole. So we, we all know that this AI is very powerful. Everyone's way of working and, and living their life is gonna be fundamentally changed by this AI technology. But it can be good or can be very risky, right? And it can, if it happens too fast, then I feel like a lot of people would be, will, will lose their job.
So, so I totally agree that AI in the long run can be a very beneficial thing for the society. But in the short run, in the short term, we probably want to be more conservative on pushing it forward. I think the whole human society needs to be more prepared on the impact it's gonna given to us. And we, we probably need [00:54:00] more regulations and we, we need more, like better.
Technology tools to control the like negative impact of ai. So I think that's, that's a major thing that's stopping AI from being more powerful which I think is good. So I think it, it takes collective efforts for everyone who is involved in this, this AI wave. No matter if for, for, for people like us, we are all like AI professionals or for just random person on the street.
They have very little knowledge of ai. I think we should, for we, we should be more like, pay more attention to this particular issue. I
Louis Bouchard: completely agree. And speaking of the long run, how do you see AI evolving over the next five years or. In your mind, where will we be at in five
Felix Tao: years? Five year, five years is probably not gonna be a huge difference.
I think of in five years, [00:55:00] probably two things that's gonna happen. One is the AI's reasoning ability is gonna be much better. So they can be totally above average, average human in terms of way to analyze and the reason to solve complicated problems. And the second thing is gonna happening, it's gonna happen, is they are gonna be able to handle more signals nowadays, mostly handle the tax data and respond by tax data as well.
But in the future, they will be able to absorb, to get like visual data voice and all these kind of different signals, different sensories that humans have. And then they can respond not only by text output, but also by some actions. By, by different type of types of ways to, to deliver information to, to the end user.
That's what's gonna happen I think, in five years. But if we think it's for a more [00:56:00] long-term perspective I, I believe like all the digital service is gonna be changed by ai. So I think AI copilot or AI agent is the new form of software in the future. So every software would be in the form of agents and everyone would be able to have a army of agents that they can leverage to help them finish a lot of things.
That's probably happening not very far, five, eight years. Yeah,
Louis Bouchard: that would be really cool. Mm-hmm. And about just the language model part. We've seen the big jump from G PT three to chat G P T and just g PT two to jet chat to g PT three as well. But would you, you, would you agree that we may have done, for example the, the per rule with whatever percentage, but like the GT three to chat G P T was [00:57:00] 20% of the work to get 80% of the results, but then the final 20% will require 80% of the work.
So like do you think the progress will slow down compared to G P T three, to G P T to chat G P T for the next big step? Or do you believe we we still will improve quite a lot in the following
Felix Tao: years? I think it's a very interesting question if you truly look into what Open Air has done over the past few years, right.
I think they are still. Trying to scale it up. Mm-hmm. And they still believe that there's a lot more can be mined from scaling the model to the next level. So my, my opinion on this is in terms of large language model itself, in the next five to three to five years, we can still make huge progress to make it make them more [00:58:00] intelligent, to make them to handle like longer context and just in general, better and more powerful.
I don't think, I don't see it slowing down, but I do believe that there is definitely some limitations on large line model itself. So we, we needed to build something, build a framework around it to unleash the power more so from that front We would see many comp, many companies many researchers to make it more autonomous, to make it more like like you said, self-teaching and to make it more powerful to, to connect to the external world and to be able to use external tools and to make it more like adaptive.
Adaptive as you use it. So all these kind of things are like a different layer of innovations on top of large language models and combining these two together because it's, you just [00:59:00] multiplies by these different factors of innovation. Right. I can see in five to 10 years, the whole AI landscape is still growing like very, very fast.
It's gonna be as fast as the past five years. Yeah.
Louis Bouchard: Even more. Yeah. That's super exciting. Yeah. Well, first I will recommend. Everyone listening to check out mine voice and mine os I think it's a really good product and it's super promising. Really cool. And I'm excited about anything, everything related to agent and, and a bit scared, but I hope, I hope it'll go well.
Do you have anything you'd like to share to the audience in terms either of mine, os or your personal projects?
Felix Tao: Yeah, it is I, I can share a little bit on mine os So mine os is currently still close the beta product. So we are currently experimenting this product with like around 500 to 1000 pilot users.
It's, it's not hundred percent ready yet, but we are iterating, [01:00:00] it's very fast, so it's probably gonna be ready within two months so it can be used by anyone in the world. So hopefully at that time it can help you. A lot and and if you are very interested in using the close beta version of mine os please go to minus versus AI and apply for trial use.
And you can, you can test this immature version and give us your valuable feedback. That will be very much appreciated.
Louis Bouchard: Awesome. Thank you very much for your time. It was super valuable and insightful. I, I really enjoyed discussing large language model and just my verse in general. I think it's a, as I said, it's a very interesting topic to challenge.
It's basically research, but like applied research, so that's amazing. And just like in research, it's super iterative and you will just keep learning and improving. So it's [01:01:00] really cool. Yeah, so thank you very much for your time. I really appreciate it.