The Best Image-to-3D AI to date: Neuralangelo
Here's the best Images-to-3D AI to date, and how it works…
Watch the video
A year ago, I made an article about a revolutionary approach called Instant NeRF. Instant NeRF is a fantastic model by NVIDIA able to take pictures and turn them into amazing 3D scenes in very little time. Think of it as an app you could use for easily creating super realistic models of any room or object for video games and other applications. It’s just awesome. Instant NeRF was quite a game changer and had a lot of potential, but the results were not perfect. The generated 3D models aren’t as crisp as reality is, and we often lack detailed structures of real-world scenes. They definitely look like AI-generated objects that are a bit cartoonish.
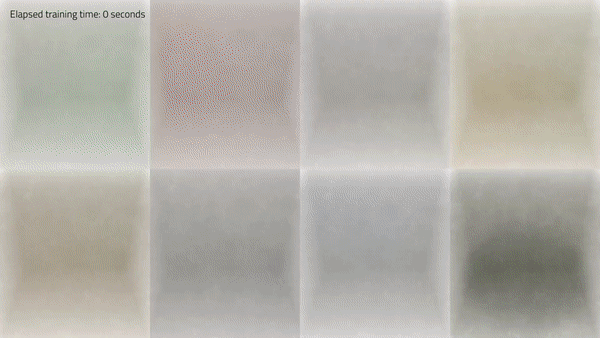
A year later, NVIDIA now releases a new approach based on Instant NeRF with much better fidelity for surface structures, beautifully called Neuralangelo. NeRF is an approach to reconstructing a real object in a virtual environment from a series of images or videos. Instant NeRF brings the typical approach to work from hours to just a few seconds while also improving the results’ quality. Neuralangelo tackled the fine-grain quality weakness by aiming to improve AI-generated 3D objects’ surface quality not only at a high level when we see the whole object and want it to look realistic but also when we look more closely and find that it’s actually not that realistic.
By the way, this great paper was published at CVPR, which I will be at in-person this year, so if you are also going, please do reach out to meet or take a coffee! I’ll also record some podcast episodes, which you will be able to find on Spotify or Apple podcasts! If you enjoy long-form content around AI, go give it a look, or rather a listen, it’s also called What’s AI by Louis Bouchard!
Let’s get back to Neuralangelo.
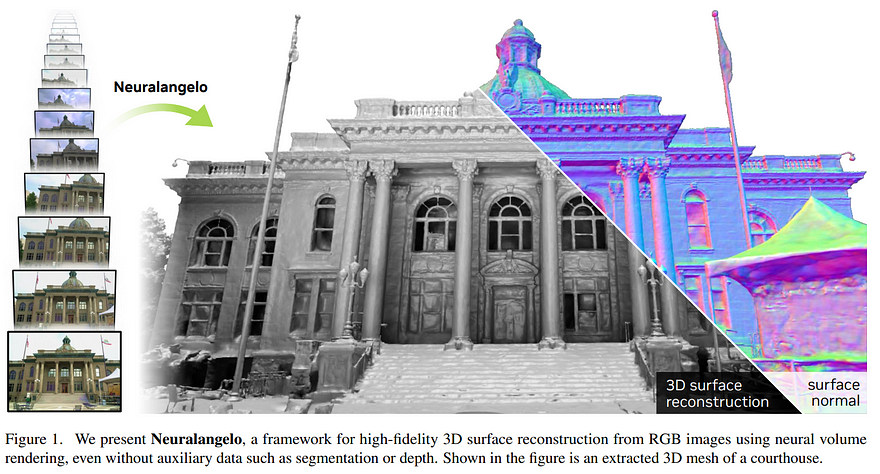
What did they do to improve the Instant NeRF approach exactly? If you are not familiar with Instant NeRF or Instant NGP, the previous NVIDIA paper, I strongly invite you to read my article about it and come back right here. I’ll wait for you before continuing, I promise. Quickly, I mainly want to remind you that Instant NGP works with hash grids encodings, which is basically a way to prepare the data from our images and camera viewpoint to feed it to our neural network for the reconstruction of the model.
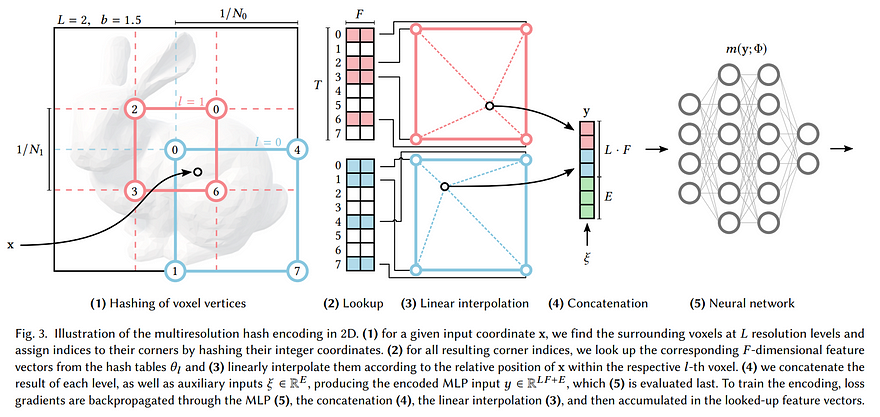
Great, now that you know about Instant NGP, let’s look at the two key differences in improving the results with Neuralangelo working on this specific hash grid encoding technique.
The first improvement is their numerical gradients for computing higher-order derivatives as a smoothing operation. Here, they are working with hash grids to represent all 3D locations along the camera view directions. As I mentioned, hash grid encoding is just a way to represent the same data that we have along our camera ray using fewer floating points and memory access operations, and it’s way more efficient. This also has the bonus of allowing the final network to be much smaller since the input we feed it, so all our data from our images and camera viewpoints, are much simpler. But we go through all that in my Instant NeRF article. Here, the important difference is that they will optimize this hash grid encoding by training it using numerical gradients instead of analytical gradients. The difference is that our numerical gradient is basically an approximation of our strictly calculated analytical gradients following rules and proper math. If our approximation is good enough, we can save lots of effort!
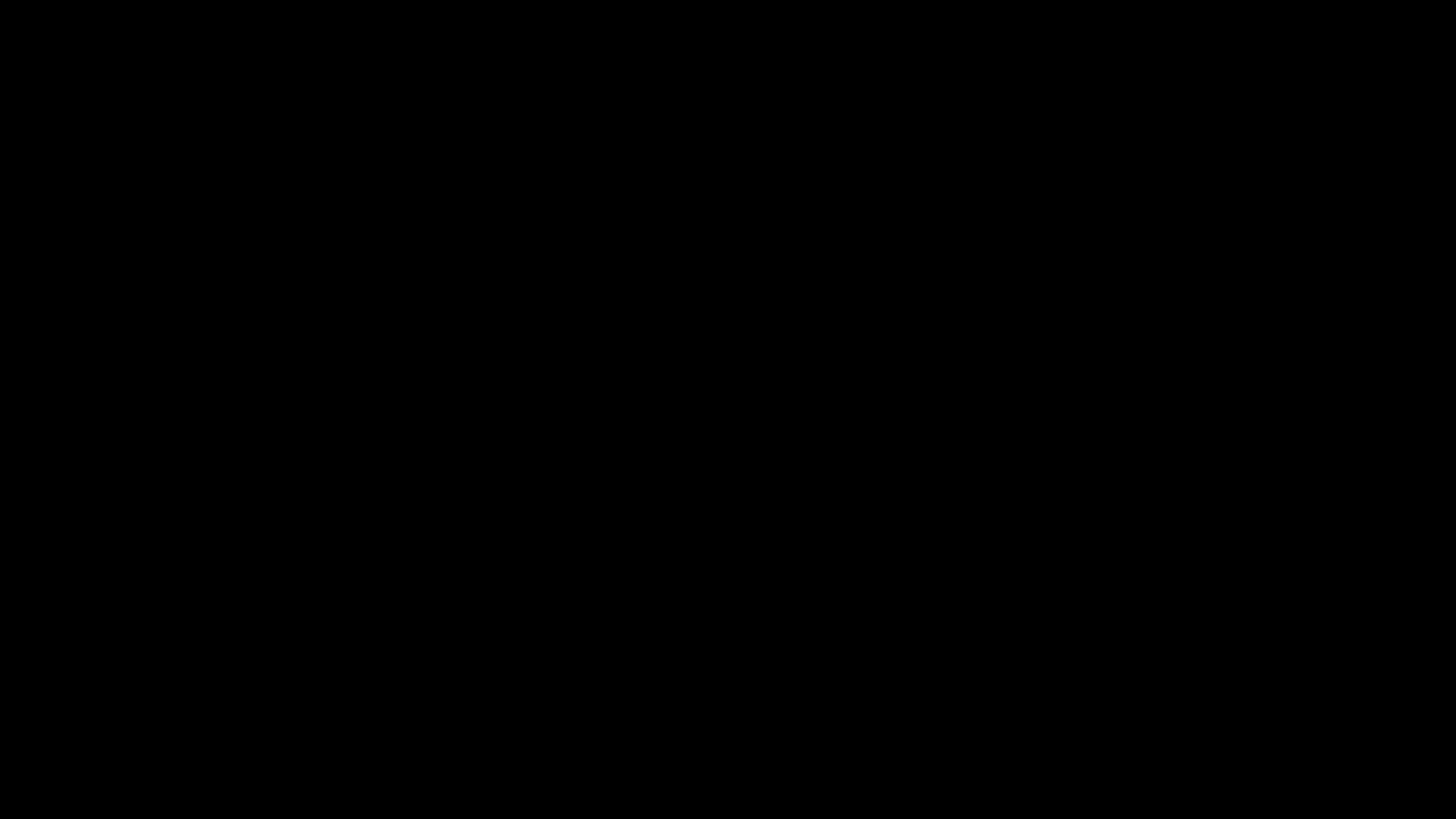
It means that if we put a large step size for the updates of the parameters during training, so asking the network to greatly change its weights would allow it to search for more information outside of its current grid cell and communicate with the grid cells around it. Something that is not possible with analytical gradients. It allows hash entries of multiple grids and an update of all their parameters simultaneously. It basically takes and blends information from more points in the encoded version of our images and camera viewpoint, which helps it create a smoother input for our upcoming network to generate the 3D model. Here, a smaller step size, smaller than the grid size of our hash grid encoding, would make it so that the analytical gradient and the numerical gradient are the same. This first step is basically to improve the consistency and smoothness of the overall input data we feed to our final network reconstructing the 3D model.
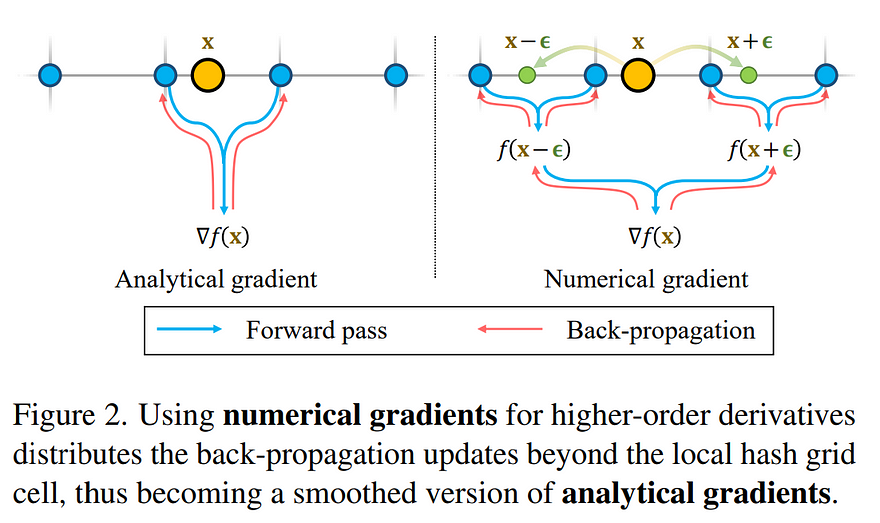
The second key difference or improvement is a coarse-to-fine optimization on the hash grids controlling different levels of details. This simply means that they will progressively decrease the step size we just talked about for calculating those numerical gradients. So optimizing this process for visual quality. As we saw, a small step would be just like calculating the analytical gradient, whereas a large step would communicate to more grid cells, so using more information and updating more parameters. So giving a larger step size relates to talking with more information and, thus, a broader view of the scene. This iterative step size decrease allows the network to first focus on the smoothed version of the scene, producing a rough draft with great overall shapes and progressively refining it through finer and finer updates through smaller step sizes, which we then feed to our network, an MLP or multi-layer perceptron, for finally guessing our colors and geometry values for the 3D model just like Instant NeRF, as we see here on the bottom right.
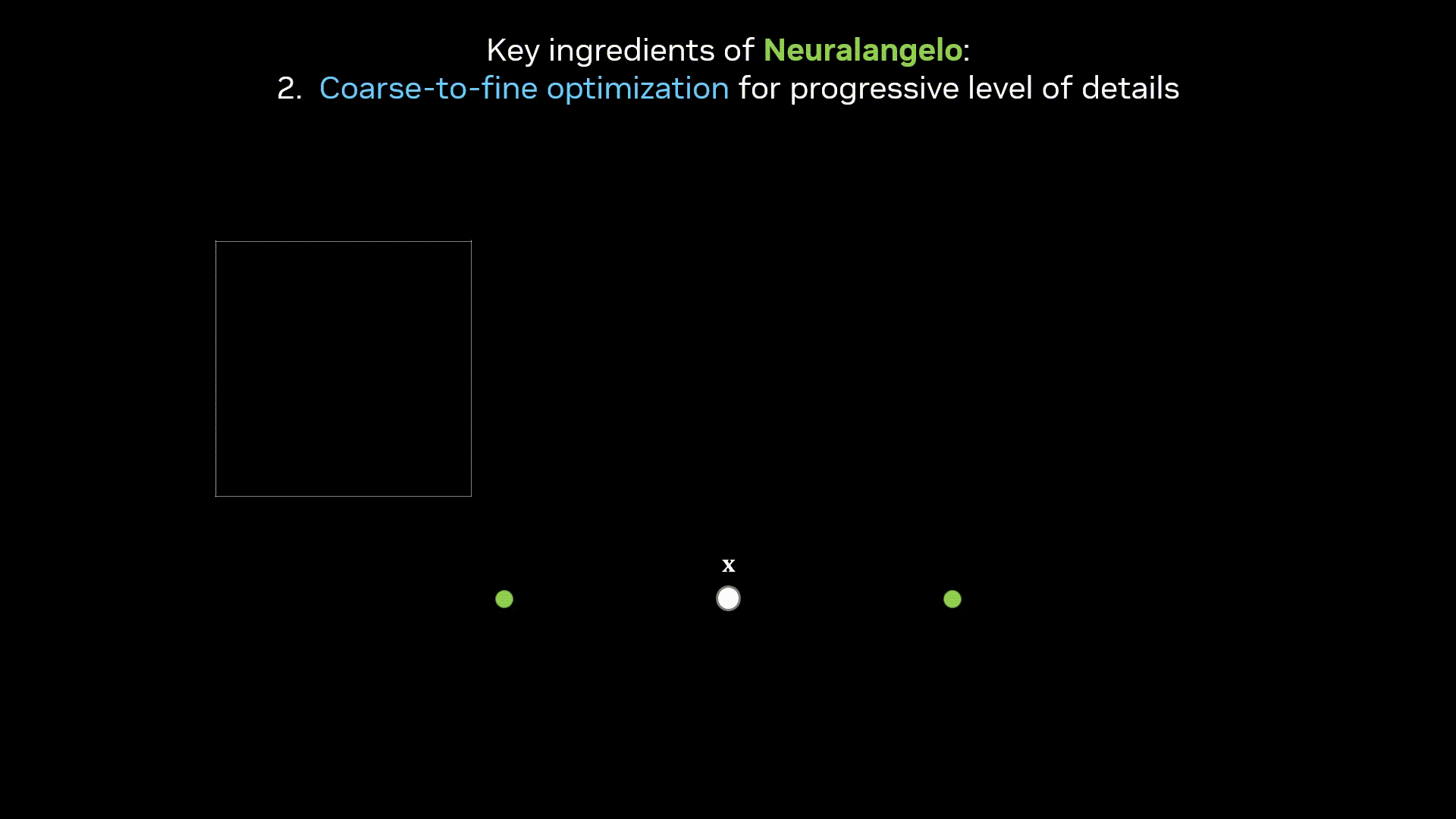
This is a similar concept as with generative models using either attention blocks or convolutions, where we first work on the broad image and iteratively get deeper into the network to work on the fine-grain details. Focusing on the broader view with a large step size produces more consistent and continuous surfaces, and switching our focus to the more fine-grain view through a smaller and smaller step size avoids smoothing the fine details we want to keep, finding the perfect balance.
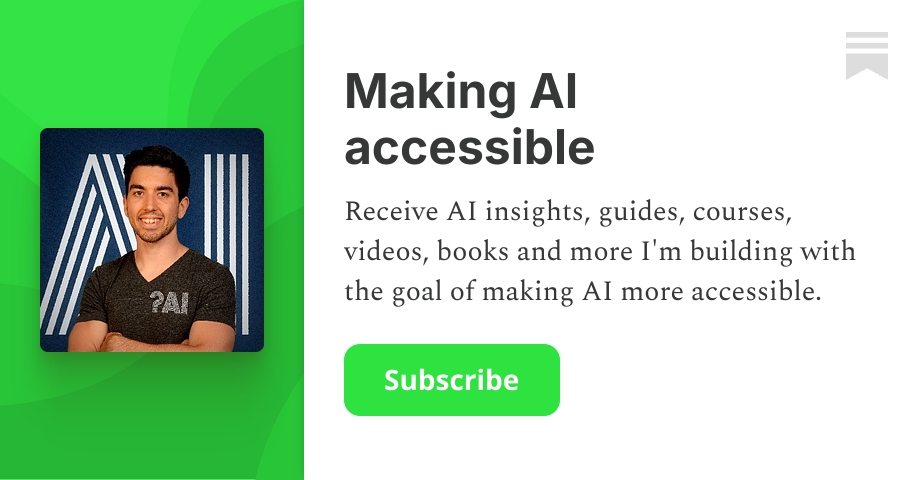
The quality of the 3D models produced is just extraordinary, even for super large scenes, and I’m excited to see it being used for real-world use cases or a future version of it. Of course, it’s not perfect. The authors mention that Neuralangelo struggles compared to previous approaches when the scene is highly reflective, like here, where Neuralangelo misses the button structures and eyes.
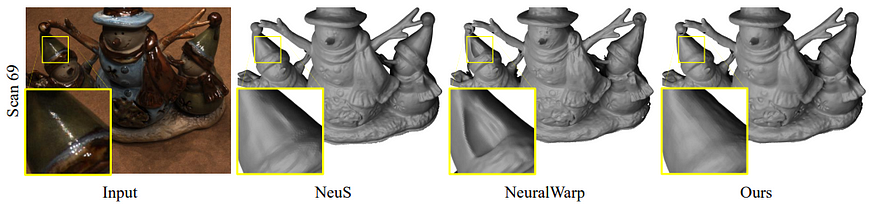
As always, this was just a quick overview highlighting this great paper published at CVPR 2023. I definitely invite you to read it for more details and subscribe to the channel if you enjoyed it and would like to stay up-to-date with new AI research like this one. I also invite you to check out my podcast on Spotify or Apple Podcasts called What’s AI by Louis Bouchard for more long-form amazing discussions with experts in the field. I have an amazing episode coming up shortly with an impressive Deepmind researcher behind the Google Map time and traject prediction algorithm!
Thank you for reading, and I will see you next time with another amazing paper!
References
- Li et al., CVPR 2023: Neuralangelo, https://research.nvidia.com/labs/dir/neuralangelo/paper.pdf
- Project page with more results: https://research.nvidia.com/labs/dir/neuralangelo/
- Instant NGP article: https://www.louisbouchard.ai/nvidia-photos-into-3d-scenes/
- The podcast: https://open.spotify.com/show/4rKRJXaXlClkDyInjHkxq3