Gen-1, the future of storytelling?
Turn mockups into videos automatically!
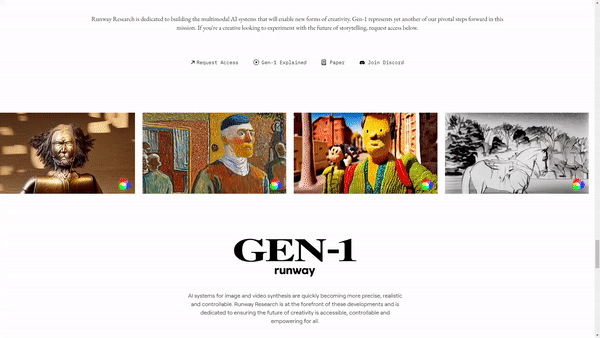
Watch the video!
The company behind one of the most popular image generation models you certainly know, Stable Diffusion, is taking it a step further.
Instead of tackling images and producing incredible results, they are now onto the next challenge: movies.
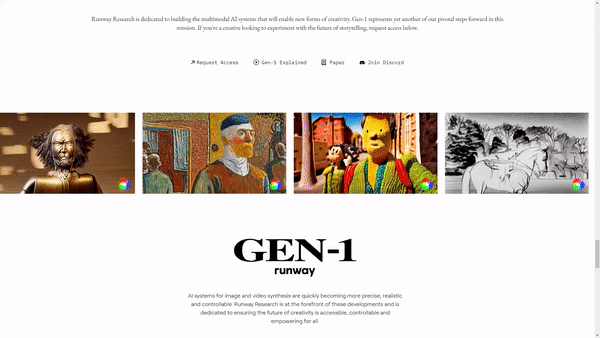
More precisely, their new model called GEN-1 is able to take a video, like this subway wagon, and apply a completely different style onto it, just like that…
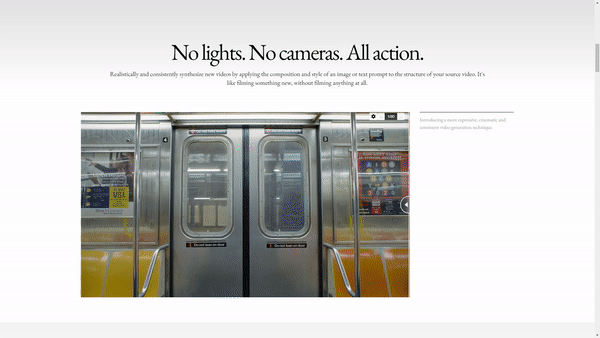
The model is still a work in progress and has many flaws, but still does a pretty cool style transfer from an image into a video, something that would’ve been impossible a few years or even months ago.
Even cooler, it also works with text. You can just say something like, “A dog with black spots on white fur.” And it will change this dog into one that fits your need!
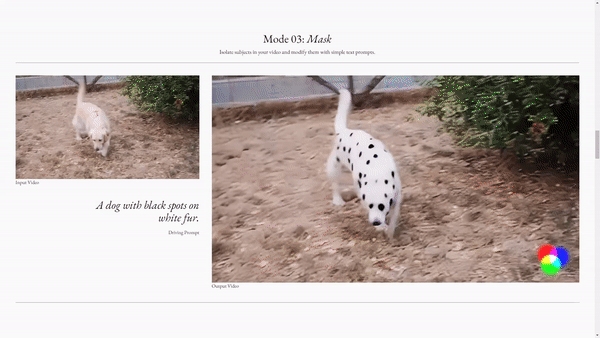
As I said, it is definitely a work in progress. We can see lots of artifacts and weird behaviors, just like Stable Diffusion or ChatGPT. We haven’t solved the problem yet. Still, take a second to look at that. What it had was only a sentence, and yet it could understand, from this video alone without any insights, where the dog was at all times and that it had to put whiter fur as well as black spots only there and nowhere else. It automatically isolated the subject, edited it, and put it back onto the video. This is impressive.
They call this approach “video-to-video”, and there are even more use cases than this stylization that we’ve seen like taking mock-ups and turning them into stylized and animated renders automatically. There are even more possibilities to do with video inputs. Just the mock-up one makes me think of all the cool ideas we could create super easily, which, as they say, could definitely be “the future of storytelling”. I’m pretty excited to see what it will look like in a few months. By the way, you can request access to the model on their website. I added all the links in the description below.
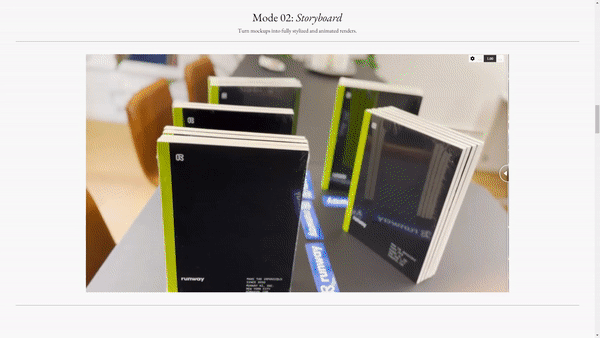
But what’s even cooler and more important than looking at the results we have is how it works…
This is where you say, “but wait, couldn’t we just use stable diffusion on all frames of a video, and that’s it?” Well, yes and no. If you do that, you need even more training data to have good results, and it has really high chance of just not being able to produce realistic results, basically having weird jumps and teleportations between frames. Still, this is a pretty good starting point and is exactly what they did.
They start from the stable diffusion model. I already covered this model when it came out, so I invite you to watch the article I made about it to learn more about the diffusion process and how this one works specifically.
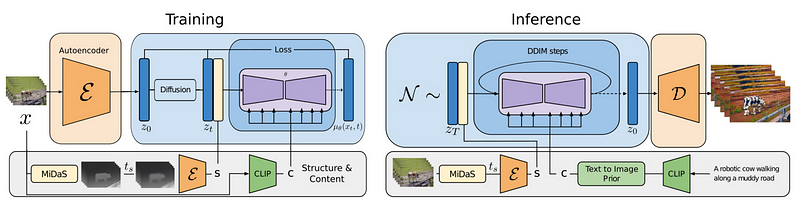
So we have a fully trained image generation model based on the diffusion process. It can already take either images or text as inputs to guide a new image generation. Here, you see the complete architecture illustrated as a training and inference separation, but note that the main difference is the use of an encoder to process an image you want to modify and a decoder to generate your final image, as we’ve discussed in my stable diffusion video. Now, how can we make it work with videos instead?
As we said, we cannot simply take all the frames and process them one by one to transform a video, or else it will look like this…
Instead, we need to adapt the architecture with some sort of understanding of time and how objects in the video evolve over time so the model can learn how to replicate this process. How can we do that?
For the problem of consistency across frames, they describe their approach to splitting a video into content and structure. Structure would be all the shapes, objects or subjects of the video as well as their movements over time. You can see now that formulating the problem like this becomes much easier than editing videos; we now strictly need to edit content and keep the structure the same.
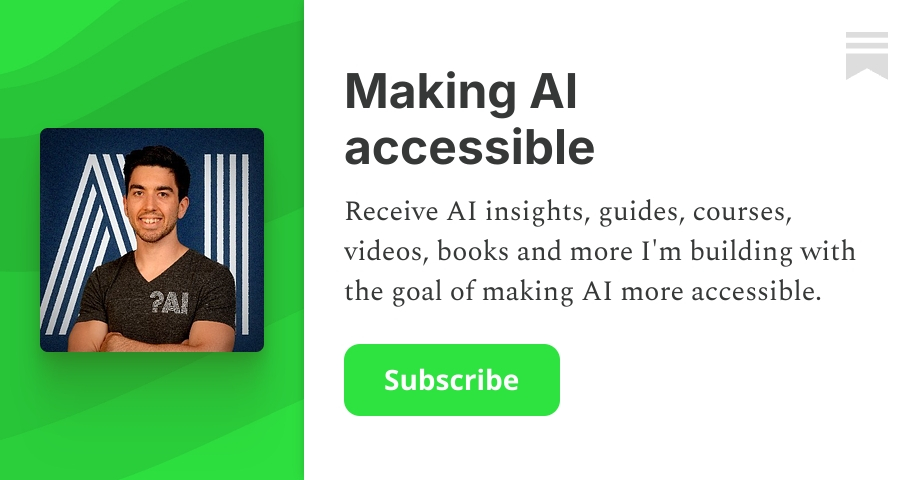
This means the video we want to transform will only be used as structural information, and the text prompt or image sent as a style to apply will only be used for the content part. This is done using two sub-models. We initially send the video into the first sub-model called MiDaS, a pre-trained model able to extract depth maps that we get our structural information from. Basically, a depth maps contains all the shapes, depths, and information we want to keep from the video.
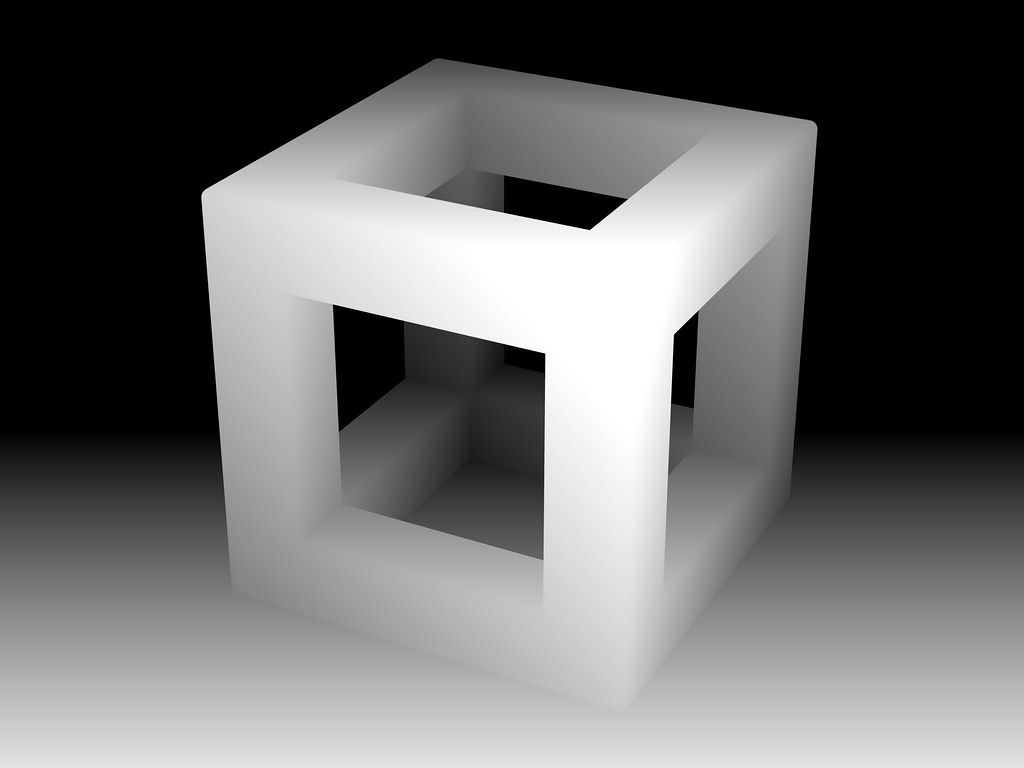
This step will remove all content-related information from the video automatically, which isn’t valuable if we want to transfer its style and allows the model to focus on structural information. Finally, we use another model called CLIP that will extract our content information. CLIP is often used to extract meanings from either text or images since it was trained on both to find how best to compare an image with its caption. This means it is pretty good at “understanding” what is happening in an image or sentence, which can also be seen as content or image style.
You now have your content and structural information to send the same diffusion architecture as stable diffusion, but adapted for videos with an extra dimension, to finally generate our new video! For those who want the extra details, this is done by adding a 1-dimensional convolution after each convolution from the image model to add an extra dimension for time. They also add positional encodings to know when are each frame and help the model understand the video. Those two additions will greatly help with consistency over time and remove the weird artifacts we mentioned.
And voilà! This is how you can take a video and transform its style completely while keeping objects and subjects relatively the same! I’m pretty excited to see what this will look like in two papers down the line, as one would say.
Limitations:
- Still has some inconsistencies across frames but much better than previous approaches
- Do not completely respect the structural information
- Generated video is not fully realistic. Has the same artifacts as stable diffusion
Of course, this was just an overview of the new Gen-1 model by Runway. I invite you to read their paper for a deeper understanding and request access to try it for yourself. I hope you’ve enjoyed the article, and I will see you next time with another amazing paper!
References
►More video examples and request access here: https://research.runwayml.com/gen1
►Esser et al., 2023: https://arxiv.org/abs/2302.03011
►My Newsletter (A new AI application explained weekly to your emails!): https://www.louisbouchard.ai/newsletter/