The AI Monthly Top 3 - November 2021
The most interesting November's AI breakthroughs with video demos, short articles, code, and paper reference.
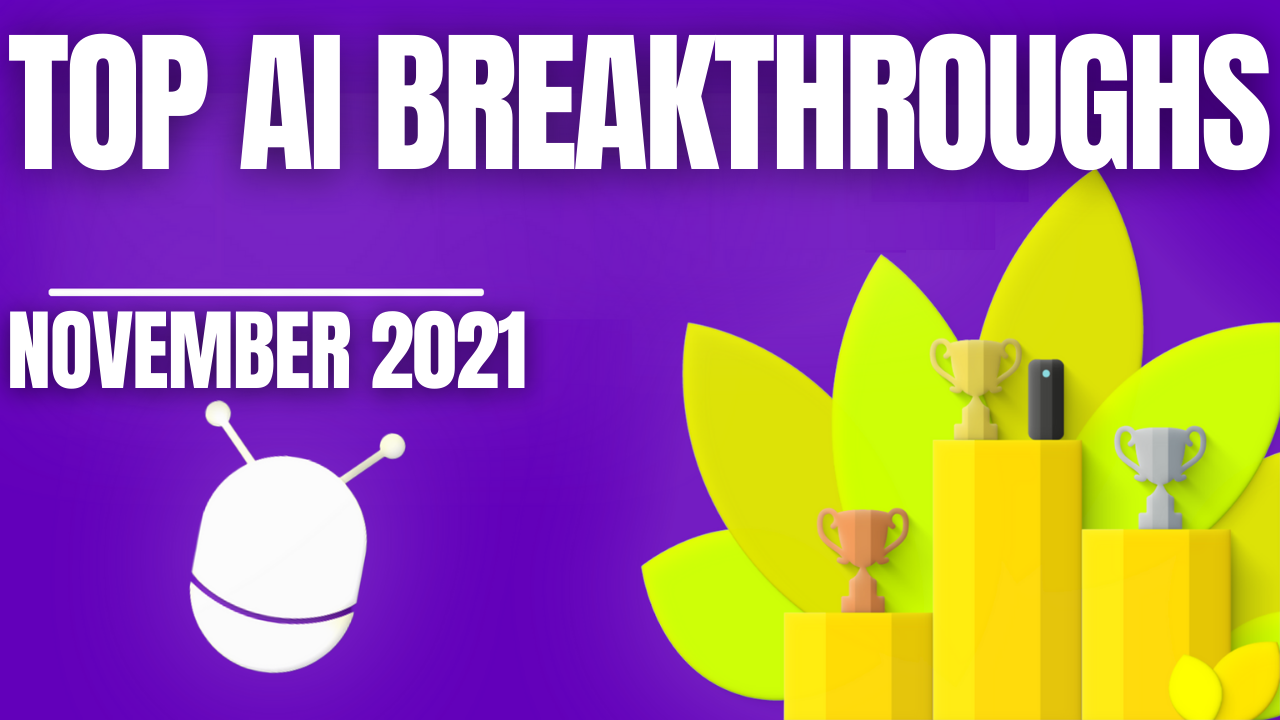
Here are the 3 most interesting research papers of the month, in case you missed any of them. It is a curated list of the latest breakthroughs in AI and Data Science by release date with a clear video explanation, link to a more in-depth article, and code (if applicable). Enjoy the read, and let me know if I missed any important papers in the comments, or by contacting me directly on LinkedIn!
If you’d like to read more research papers as well, I recommend you read my article where I share my best tips for finding and reading more research papers.
Follow me on Medium to see this AI top 3 monthly!
Paper #1:
StyleCLIPDraw: Coupling Content and Style in Text-to-Drawing Synthesis [1, 2]
Have you ever dreamed of taking the style of a picture, like this cool TikTok drawing style on the left, and applying it to a new picture of your choice? Well, I did, and it has never been easier to do. In fact, you can even achieve that from only text and can try it right now with this new method and their Google Colab notebook available for everyone (see references). Simply take a picture of the style you want to copy, enter the text you want to generate, and this algorithm will generate a new picture out of it! Just look back at the results above, such a big step forward! The results are extremely impressive, especially if you consider that they were made from a single line of text!
Watch the video
A short read version
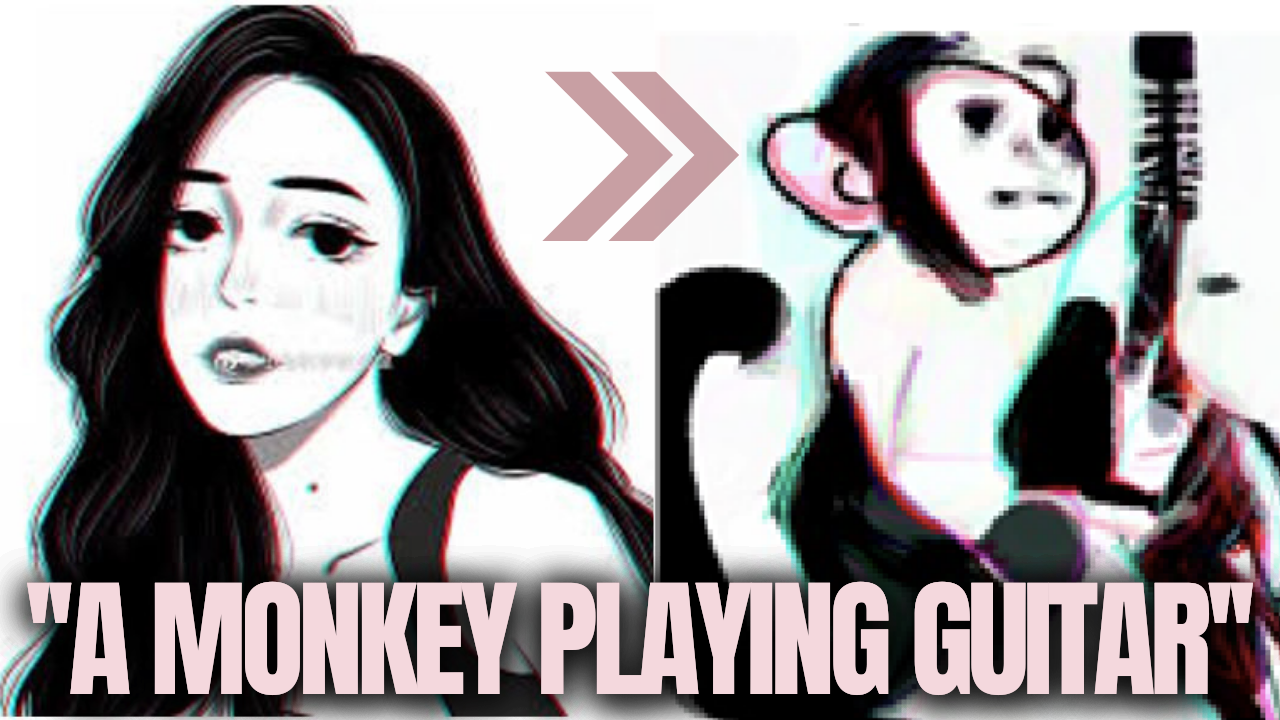
- CLIPDraw code: https://colab.research.google.com/github/kvfrans/clipdraw/blob/main/clipdraw.ipynb
- StyleCLIPDraw code: https://github.com/pschaldenbrand/StyleCLIPDraw
- StyleCLIPDraw Colab: https://colab.research.google.com/github/pschaldenbrand/StyleCLIPDraw/blob/master/Style_ClipDraw.ipynb
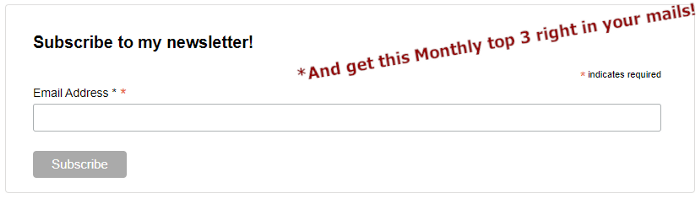
Paper #2:
SwinIR: Image restoration using swin transformer [3]
Have you ever had an image you really liked and could only manage to find a small version of it that looked like this image above on the left? How cool would it be if you could take this image and make it twice look as good? It’s great, but what if you could make it even four or eight times more high definition? Now we’re talking, just look at that.
Here we enhanced the resolution of the image by a factor of four, meaning that we have four times more height and width pixels for more details, making it look a lot smoother. The best thing is that this is done within a few seconds, completely automatically, and works with pretty much any image. Oh, and you can even use it yourself with a demo they made available…
Watch the video
A short read version
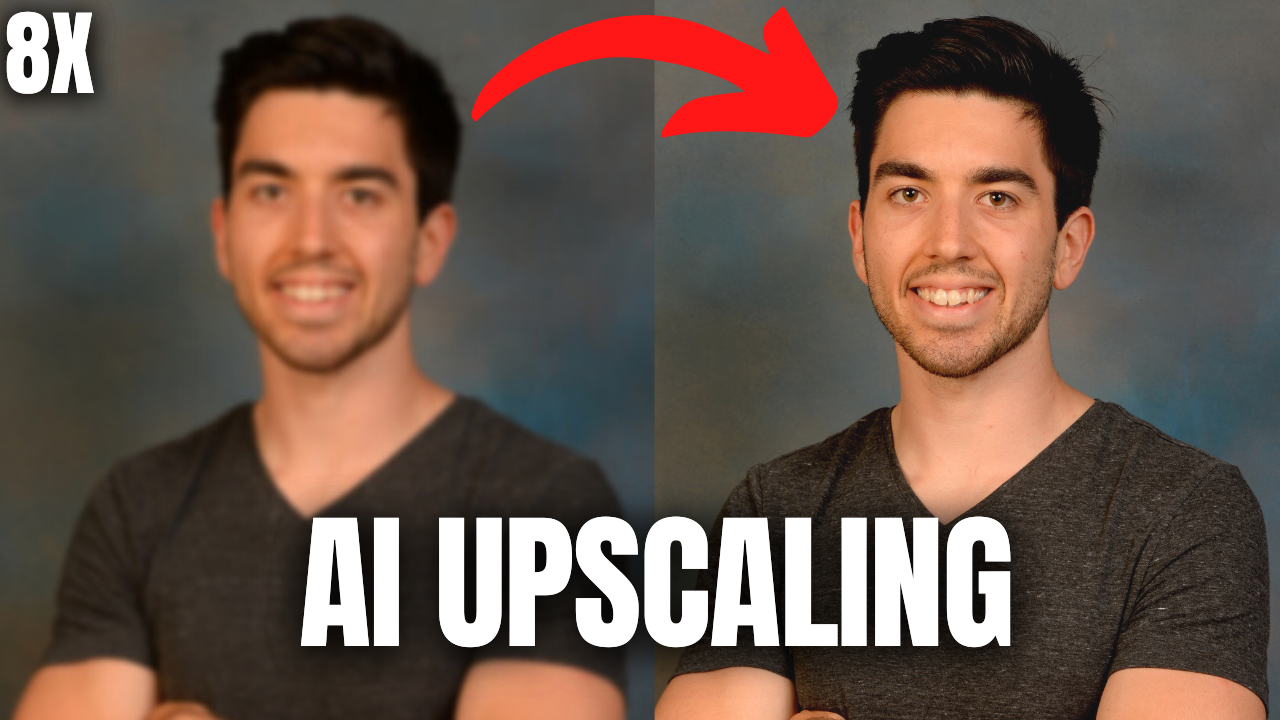
Paper #3:
EditGAN: High-Precision Semantic Image Editing [3]
Control any feature from quick drafts, and it will only edit what you want keeping the rest of the image the same! SOTA Image Editing from sketches model based on GANs by NVIDIA, MIT and UofT...
Watch the video
A short read version
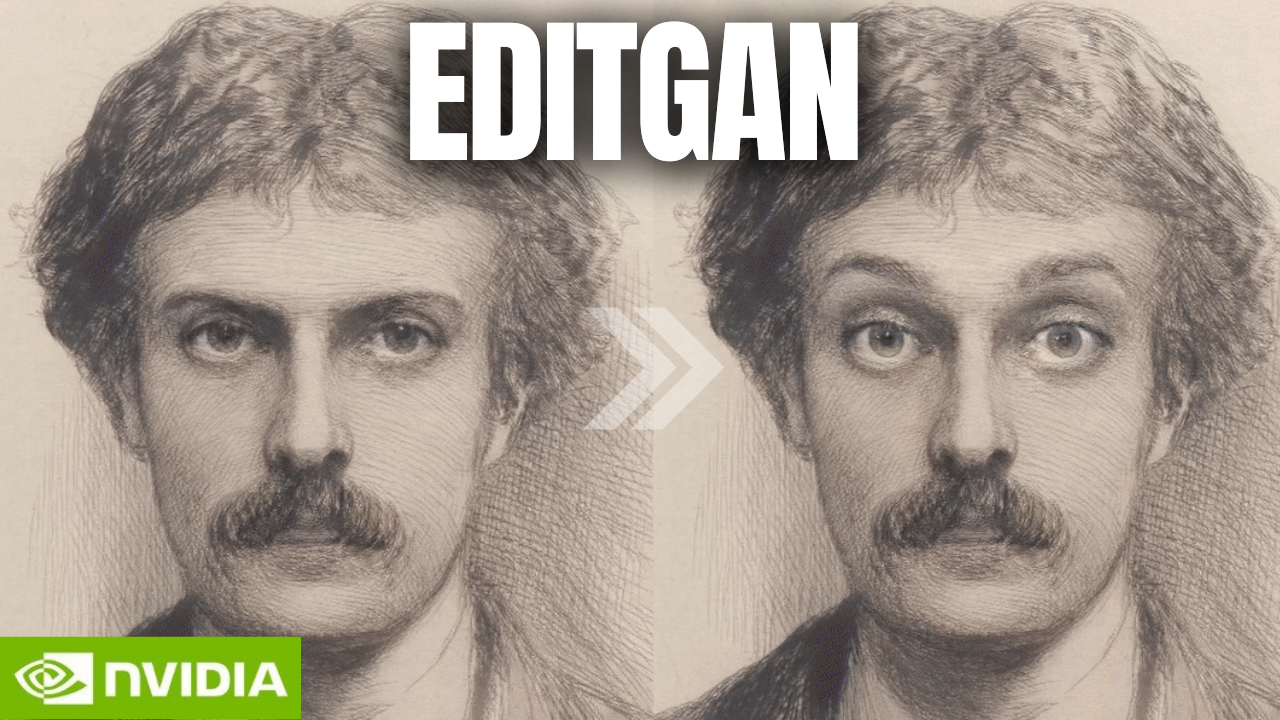
Code (coming soon): https://nv-tlabs.github.io/editGAN/
If you like my work and want to stay up-to-date with AI, you should definitely follow me on my other social media accounts (LinkedIn, Twitter) and subscribe to my weekly AI newsletter!
To support me:
- The best way to support me is by following me here or on Medium or subscribe to my channel on YouTube if you like the video format.
- Support my work on Patreon.
- Join our Discord community: Learn AI Together and share your projects, papers, best courses, find Kaggle teammates, and much more!
- Here are the most useful tools I use daily as a research scientist for finding and reading AI research papers… Read more here.
References
[1] CLIPDraw: Frans, K., Soros, L.B. and Witkowski, O., 2021. CLIPDraw: exploring text-to-drawing synthesis through language-image encoders. https://arxiv.org/abs/2106.14843
[2] StyleCLIPDraw: Schaldenbrand, P., Liu, Z. and Oh, J., 2021. StyleCLIPDraw: Coupling Content and Style in Text-to-Drawing Synthesis. https://arxiv.org/abs/2111.03133
[3] Liang, J., Cao, J., Sun, G., Zhang, K., Van Gool, L. and Timofte, R., 2021. SwinIR: Image restoration using swin transformer. In Proceedings of the IEEE/CVF International Conference on Computer Vision (pp. 1833–1844).
[4] Ling, H., Kreis, K., Li, D., Kim, S.W., Torralba, A. and Fidler, S., 2021, May. EditGAN: High-Precision Semantic Image Editing. In Thirty-Fifth Conference on Neural Information Processing Systems.